Abstract
Orientation: In light of the global financial instabilities, investors and risk analysts need extreme risk management tools to help them accurately monitor and reduce market exposure in an investment portfolio.
Research purpose: The main aim of the study was to apply extreme value theory results to quantify the extreme downside risk and upside risk of the South African Financial Index (J580).
Motivation for the study: Financial markets have been characterised by significant instabilities caused by occurrence of extreme events. This means there is a need to develop proper risk management models that can accurately assess these extreme events.
Research approach, design and method: The peak over threshold approach was used to obtain the excess returns over the threshold. The generalised Pareto distribution (GPD) was fitted to the excess returns over the threshold to estimate the parameters, which were used to quantify the downside and upside risk in the form of value at risk and expected shortfall.
Main findings: The findings indicate that the upside risk of the Financial Index (J580) outweighs the downside risk.
Practical/managerial implications: These findings would be important for hedging purposes, investment decision-making and help risk analysts to monitor the exposure of market risk and protect their investment portfolios accordingly.
Contribution/value-add: This article will contribute to empirical evidence of the research into the behaviour of the extreme returns on the Johannesburg Stock Exchange. The GPD model formulated will be used to assess tail-related risk.
Keywords: extreme value theory; peak over threshold; generalised Pareto distribution; financial index (J580); value at risk; expected shortfall; downside risk; upside risk.
Introduction
Orientation
In the study and practice of financial risk management, the value at risk (VAR) and expected shortfall (ES) metrics are the most widely used risk measures. Proper estimation of VAR and ES is necessary in that they need to accurately capture the level of risk exposure (downside and upside risk) that the firm or portfolio is exposed to. If a business entity overestimates the risk level, then the firm will unnecessarily set aside capital to cover the financial risk when the capital could have been utilised elsewhere. Investors and risk analysts have become more concerned with events occurring under extreme market conditions.
Extreme value theory (EVT) application is found in many fields where extreme events may occur, including hydrology (Katz, Parlange & Naveau 2002), insurance (McNeil 1997) and finance (Gencay & Selçuk 2004). Extreme value theory provides a solid framework for estimating the behaviour of extreme events and performs better than other approaches in terms of predicting unexpected extreme movements as it focusses on the tails of the distribution (Longin 2000). In this study, EVT is used to estimate financial risk measure in the South African Financial Index. Extreme value theory provides appropriate distributions to capture extreme events. The generalised Pareto distribution (GPD) of the EVT is used to estimate financial risk measures (downside and upside risk) in the form of VAR and ES for the index. The measures are very useful because they are good at assessing the likelihood and impact of a financial crisis.
In the last two decades, the phenomenon of the occurrence of rare extreme events seems to be more apparent in financial markets around the world, including the South African market. The markets have been characterised by significant instabilities. This means there is a need to develop proper risk management models that can assess both the probabilities of risky events in normal market conditions and also rare financial events, like the global financial crisis of 2007–2008. The behaviour of the tails of the South African Financial Index (J580) is considered, with a specific focus on the use of GPD to estimate tail-related risk with an aim of providing a modern risk management model that will help in determining the downside risk and upside risk, in the form of VAR and ES.
Research purpose and objectives
The objectives in this article are therefore as follows:
- to fit the GPD model to the monthly J580 Index data
- to estimate downside risk and upside risk using the VAR and ES for different levels of statistical confidence.
This study focusses on modelling extreme events using the GPD and contributes to empirical evidence on research into the behaviour of the extreme financial returns of the J580 Index on the Johannesburg Stock Exchange (JSE) and will help risk analysts to monitor the exposure of market risk. This study is organised as follows: the next section presents a literature review, followed by research models. Next the results and discussion are provided and finally the conclusion and areas for further study.
Literature Review
The literature is full of examples of financial risk associated with rare but high impact events: De Dieu, Mwita and Mung’atu (2014), Vee, Gonpot and Sookia (2014), Kiragu and Mung’atu (2016), Nortey, Asare and Mettle (2015) and many others. Magnou (2016), Vladimir et al. (2016) and Zhaoa et al. (2010) are discussed in this study. Magnou (2016) modelled financial risk in the Uruguayan Pension Fund using EVT. The researcher assessed the probability of rare and extreme events as an important issue in the risk management of financial portfolios. In this study, the researcher used the peak over threshold (POT) approach of the GPD model, which gives information on tail distribution of financial losses. The researcher concluded that the GPD model is useful for assessing the size of extreme events. Value at risk approaches, based on the assumption of the normal distribution area, overestimate low percentiles (because of the smaller tail of the normal distribution) and underestimate high percentiles (because of heavy tails in the data set, which is not accommodated by a normal distribution). The researcher concluded that the GPD model is useful for assessing the size of extreme events and is easy to implement.
Vladimir et al. (2016) modelled the Russian Stock Market Index (RTSI) by an application of GPD of EVT. In their study, they utilised the GPD, which gives information on the tail distribution of financial returns. The authors applied the GPD method to RTSI daily losses from 1995 to 2009. The RTSI is a total index of the 50 largest Russian stocks. Vladimir et al. (2016) focussed on the assessment of tail-related risk measure. The authors concluded that the GPD method fits the tail distribution of financial return series and estimates extreme financial risk more accurately compared with the traditional method of risk estimation, which assumes the normal distribution. The authors also recalculated VAR using the GPD model and concluded that EVT is the most appropriate approach.
Zhaoa et al. (2010) used EVT for forecasting the impact of the market crisis in New Zealand. The authors used EVT to quantify VAR, which was then used to estimate the probability of the impact of the financial crisis. As in the study by Vladimir et al. (2016), the authors used daily returns data (2001–2008) of the Citigroup and S&P 100 Index. The authors used the GPD model, which models the tail distribution of financial returns for both losses and gains. Zhaoa et al. (2010) concluded that the GPD method is suitable for estimating the probability of impact of a financial crisis using stock and index returns.
There are numerous studies that have analysed extreme events in the financial markets resulting from the currency crises, equity market turmoil and credit defaults, including Rydell (2013), Chege, Mungat’u and Ngesa (2016) and Omari, Mwita and Waititu (2017). Loretan and Phillips (1994), Gilli and Këllezi (2006) and Onour (2010) discussed the behaviour of tail distributions of a financial return series using GPD. The potential of EVT in risk management was discussed by Diebold et al. (1998). This study will fill the gap in the literature regarding the development and formulation of extreme risk management models that accurately capture the level of risk exposure and hedge against an investment risk on the South African Equity Market using the J580 Index.
Research models
Extreme value theory best describes the extreme value distributions that are associated with financial returns. It provides a model that gives guidance on the kind of distributions that should be selected so that the parameters are estimated, which are then used to calculate VAR and ES.
The block maxima (BM) approach and the POT approach are the two main methods under the EVT model. The BM approach models data by fitting the generalised extreme value distribution to a set of block maxima data. According to Gilli and Këllezi (2006), when one block contains more extreme data values than another, the use of the POT approach for modelling the extreme events is then preferred. The POT approach is the most preferred compared to the BM approach because it uses most of the data efficiently and produces more reliable results (McNeil 1997). In this study, the POT approach was adopted.
Generalised Pareto distribution and peak over threshold
According Pickands (1975) and Balkema and deHaan (1974), the GPD is defined by the following:
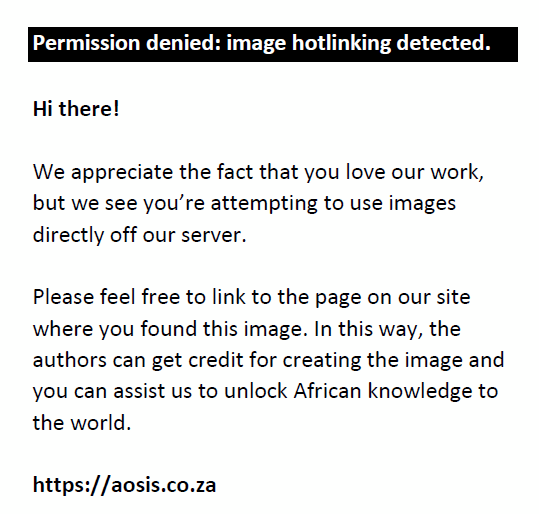
Given that x > 0 when ξ ≥ 0 and 0 ≤ x ≤ – β/ξ when ξ < 0 and β > 0, Fξ,β (x) is defined as the GPD with the shape parameter or the tail index ξ, a scale parameter β and a threshold u. The value of the shape parameter ξ shows how heavy the tail of the distribution is, with a large value indicating a very heavy tail. According to Gilli and Këllezi (2006) distributions with a tail of ξ ≥ 0 are generally suitable for modelling financial return series. The density function is given as follows:

Peak over threshold
According to Chen and Giles (2015) the POT approach is a suitable method for analysing extreme risk as it offers a parametric form for tail distribution. The POT approach uses exceedances above a specified high threshold. The GPD is fitted to the exceedances above the threshold. The parameters are estimated by the maximum likelihood estimate (MLE) method. Sample mean excess plot, shape parameter and Pareto Quantile-Quantile (QQ) plot are the methods used to help in the selection of the threshold u (Chen & Giles 2015) and are adopted in this study.
Excess distribution
For a random variable X with a density function F, the excess distribution above a certain threshold u is defined by the following:

where x represents the size of exceedances over u. If F is denoted as the distribution function for X then we may write the following:

Fu is the conditional excess distribution function.
Risk measures (downside and upside risk)
In this study the extreme risk (downside and upside risk) is evaluated for both long and short positions held by investors. The two financial risk measures, namely VAR and ES, are considered.
Value at risk is defined as the maximum amount that will be lost over a particular holding period with a particular degree of confidence. It is generally defined as the sufficient capital to cover the losses from a portfolio over a holding period of a fixed number of days. Another measure of downside risk or the tail conditional expectation that estimates the potential size of the loss exceeding VAR is the ES. Value at risk does not capture all aspects of market risks, such as subadditivity. Expected shortfall is a better measure of risk that is subadditive and also informs about the likely magnitude of exceedances. Expected shortfall is the expected size of return that exceeds VAR for a probability level p.
The following from Ren and Giles (2010) gives an estimate of the statistical cumulative distribution function.

where the total sample size is n, and Nu is the number of observations above the chosen threshold.
The inverse of Equation 5 (value of x in Equation 5) with a probability p gives the VAR:

For ξ < 1 the ES is given by the following:

However, VAR is not a coherent risk measure; in simple terms coherence implies that when loss distributions are altered or combined, the risk measures used still behave sensibly.
Threshold and parameter estimation
The mean excess plot
In order to adopt the POT approach, the choice of the threshold is critically important to the modelling of the tails of the distribution. The sample mean excess live plot is a graphical method that helps in the estimation of the threshold u. From the mean excess function, the sample excess function, e(u), is estimated and is defined as follows:

This function is expected to be linear and this property of linearity is then used as a basis for the estimation of u.
The Hill plot
If x1 > x2 >… … … > xn is the ordered statistic of the independent and identically distributed random variables, using k + 1 ordered statistics the Hill estimator is defined by the following:
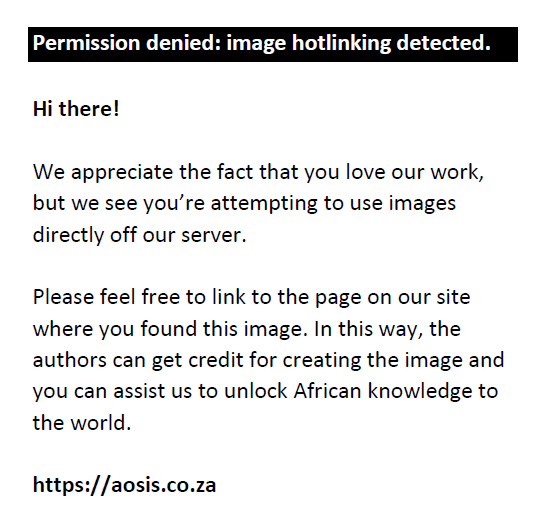
where k = k(n) → ∞ is upper order statistics (the number of exceedances), n is the sample size and the tail index, α = 1/ξ. From this graph the threshold u is estimated from the stable areas of the tail index in the graph.
Parameter estimation using maximum likelihood estimation method
The parameters of the GPD are estimated using the MLE method. According to Chege et al. (2016), the joint density function of a sample size n that is independent and identically distributed is given as follows:

where θ = (ξ, β) are the parameters of the model f(xi | θ). For the known observed variables, the parameters are given by θ = (ξ, β) for the given threshold u to be selected. The likelihood function is then given by the following:

and the logarithmic likelihood function is given by the following:

The set of the estimated parameters maximises the likelihood function, Equation 11 or 12.
Results and discussion
In this section, data analysis and findings are discussed. The data analysis is performed in the R programming environment, using the packages fExtremes, extRemes, evir and ismev. This study applied GPD of EVT in analysing extreme returns of the J580 Index to obtain shape and scale parameters that are used to estimate the upside and downside risk.
Data
The data span from 1995 to 2018, making a total of 272 monthly index observations of the J580 Index from the website https://expert.inetbfa.com. The aim of the study is to model the tail distribution of the J580 Index and estimate the tail-related risk.
A logarithmic transformation was performed on the raw data to obtain logarithmic index returns as follows:

where rt represents the monthly log returns on month t, Mt represents the monthly returns in month t and ln denotes the natural logarithm.
The results for both the right and left tails of the return distribution are generated. The right tail (negative returns) represents the downside risk and the left tail (positive returns) represents the upside risk of the empirical return distribution.
Basic statistics
The characteristics of a financial time series such as positive skewness and a large positive kurtosis were found present in this data set. Table 1 shows that monthly J580 Index returns have a skewness of 2.194 and a high kurtosis of 19.440, implying that the data is fat tailed (Omari et al. 2017). The mean is –0.835 with a small standard deviation of 0.06042. The minimum and maximum values are far apart, implying the data consists of extreme values.
TABLE 1: Monthly log returns of the J580 Index descriptive statistics. |
Testing for normality, stationarity and auto-correlation in the data set
The p = 6.903e-08 was obtained using the Anderson Darling test, which is less than 0.05: we therefore rejected the hypothesis of normality and concluded that the J580 Index return series is not normally distributed.
A p-value smaller than 0.01 was obtained using the augmented Dickey-Fuller test; therefore the null hypothesis of non-stationarity was rejected, and it was concluded that the data is stationary.
The Box Ljung (lag = 10) test revealed there was no presence of significant auto-correlation in the returns series because the auto-correlations were almost zero, indicating that the return series is independent and identically distributed.
Testing for heteroscedasticity
The Lagrangian multiplier test results indicated no presence of significant autoregressive conditional heteroscedastic (ARCH) effects in the J580 return series (chi-square = 0.1155, df = 12, p = 1). Auto-correlation and conditional heteroscedasticity tests showed that there is no existence of auto-correlation and no persistence of variance in the standardised series and squared standardised series, therefore no evidence of volatility clustering in the return series.
Determination of the threshold
In this study, both downside and upside risk measures were of interest. Therefore the negative returns were separated from positive returns and modelled separately using the GPD distribution. The upside and downside risks corresponding to the VAR and ES risk measures were estimated. The threshold for both the positive and negative returns was determined using the Pareto QQ plot, the Hill plot and the mean residual live plot. These three methods were used to select a suitably high threshold to fit the GPD to the values in excess of the threshold.
In Figure 1, a tangent line to the data points in the Pareto QQ plot will aid in determining the threshold. The line crosses the y-axis at about −3.5, implying that the threshold is 0.03 (the exponent of −3.5 is 0.03).
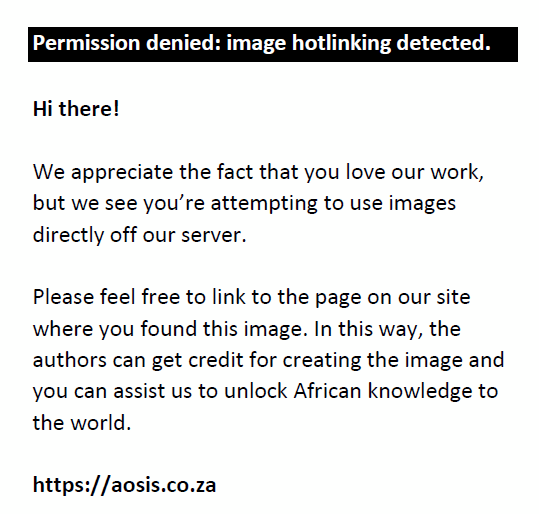 |
FIGURE 1: Pareto Quantile-Quantile diagram for determining the threshold for positive returns (left tail or gains). |
|
A Pareto QQ diagram for determining the threshold for negative returns losses (right tail) was also used. It was found that the threshold is 0.045.
The optimal threshold selected from a relatively steady area on the Hill plot should provide sufficient exceedances to the fitted GPD. In Figure 2 the order statistics range in which we expect the threshold is 57–64. This confirms a threshold estimate of 0.03.
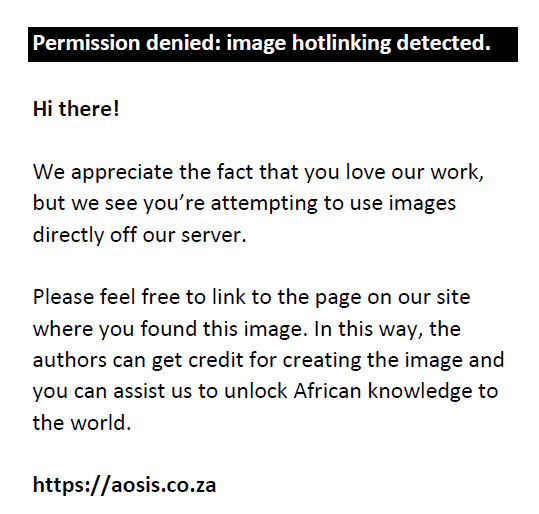 |
FIGURE 2: Hill plot for the left tail (positive returns or gains). |
|
The Hill plot for the right tail of the return series was also determined. The order statistics range in which we expect the threshold is 55–65, in which the threshold is then determined to be 0.045.
According to literature, the mean residual live plot indicates that the thresholds are supposed to lie in a particular linear positive slope range on the plot.
The threshold is confirmed to be 0.045 in Figure 3 for the right tail (negative returns). The graph becomes unstable beyond 0.07.
 |
FIGURE 3: Mean residual plot for the right tail (negative returns [losses]). |
|
Threshold values of 0.03 for the left tail (positive returns) were determined in relation to the Pareto QQ plot, Hill plot and mean residual live plot.
The left tail goodness-of-fit plots for the GPD model are shown in Figure 4.
 |
FIGURE 4: (a) Probability, (b) quantile, (c) return level and (d) density plots for the left tail (positive returns, representing the gains). |
|
The probability–probability (PP) and quantile–quantile (QQ) plots do not move away significantly from the straight line; the density plots confirms the GPD provides a good fit to the exceedances in Figure 4. There are no points outside the confidence limits for the return level plots, indicating a good fit for the left tail.
The goodness-of-fit plots for the right tail (negative returns) also indicated a good fit.
Fitting the generalised Pareto distribution using the maximum likelihood estimate method
The GPD is fitted to the excesses returns over the threshold to estimate the parameters that will be used to estimate VAR and ES. Table 2 shows the shape and the scale parameters from the fitted GPD and their corresponding standard errors.
TABLE 2: Parameter estimation using maximum likelihood estimate method for J580 Index. |
The shape and the scale parameters of the right tail are ξ = −0.06586863 and σ = 0.110349819. The shape parameter ξ < 0 means that the right tail is shorter than the tail of the normal distribution, which means that the probability of extreme losses because of a long position on the index is minimal. The right tail follows the Weibull class distribution because ξ < 0, and it has a finite upper bound, indicating an absolute maximum.
The shape and scale parameters for the left tail are ξ = 0.28252147 and σ = 0.32166984. A shape parameter ξ > 0 means that the left tail has heavier tails than the normal distribution, which can result in extreme gains occurring. The left tail follows the Fretchet family of distributions. The positive shape parameter is of interest in financial risk management.
Estimating risk measures (downside risk and upside risk)
Using the shape and scale parameter estimates obtained in Table 2, the VAR and ES are estimated. In this study we considered the right and left tails of the return distribution. The right tail represents losses for an investor with a long position on the index, and the left tail represents losses for an investor, being short on the index.
In this study negative returns and positive returns are used to determine the downside risk and upside risk respectively. Value at risk and ES are used to estimate the downside risk and upside risk (Table 3). Value at risk estimates risk exposure and ES estimates the potential size of loss exceeding VAR at a given probability level. The expected monthly gain will not exceed 15.66%, and if it does the gain will be 24.39% at the 95% level of confidence. The expected monthly loss will not exceed 14.31%, and if it does the loss will be 17.10% at the 95% level of significance. The interpretation is the same at the 99% and 99.5% levels of confidence. These findings imply that the upside risk of the J580 Index outweighs the downside risk. Therefore, it can help investors and risk managers to monitor the exposure of market risk and protect their portfolios accordingly.
TABLE 3: Risk measures of value at risk and expected shortfall for the right tail and the left tail. |
Conclusion
In this study, EVT was used to model the tail behaviour of the J580 Index for the right tail and the left tail. The POT method was used to estimate the threshold using the Pareto QQ plot, Hills plot and mean residual live plot. The POT method was favoured as it tends to utilise data more efficiently. The MLE method was used to estimate the parameters. Extreme tail risk measures were estimated at relatively high quantiles at the 95%, 99% and 99.5% confidence intervals. The VAR and ES are used for analysing the extent of potential extreme downside and upside risk of the J580 Index. These findings highlight the importance of the GPD in fitting appropriately the tails of financial time series data characterised by extreme events. The conclusion is that the upside risk of the J580 Index outweighs the downside risk. For investors and risk analysts, these risk measures would be important for hedging purposes and investment decision-making.
For areas of further study the authors recommend the modelling of extreme value dependence using copula functions.
Acknowledgements
Competing interests
The authors declare that they have no financial or personal relationships that may have inappropriately influenced them in writing this article.
Authors’ contributions
O.J. analysed the data and wrote the article, and D.C. provided the initial ideas and proofread the article.
Disclaimer
The views expressed in this article are those of the authors and not an official position of the institution.
References
Balkema, B. & deHaan, L., 1974, ‘Residual lifetime at great age’, Annals of Probability 2, 792–804. https://doi.org/10.1214/aop/1176996548
Chege, C.K., Mungat’u, J.K. & Ngesa, O., 2016, ‘Estimating the extreme financial risk of the Kenyan Shilling Versus US Dollar exchange rates’, Science Journal of Applied Mathematics and Statistics 4(6), 249–255. https://doi.org/10.11648/j.sjams.20160406.11
Chen, Q. & Giles, D.E., 2015, Risk analysis for three precious metals: An application of extreme value theory, Sauder School of Business, University of British Columbia Vancouver, B.C., Canada.
De Dieu, N.J., Mwita, P.N. & Mung’atu, J.K., 2014, ‘Estimation of extreme value at risk in Rwanda exchange rate’, European Journal Statistics and Probability 2(3), 14–22.
Diebold, F.X., Schuermann, T. & Stroughair, J.D., 1998, ‘Pitfalls and opportunities in the use of extreme value theory in risk management’, Journal of Risk Finance 1, 30–35. https://doi.org/10.1108/eb043443
Gencay, R. & Selcuk, F., 2004, ‘Extreme value theory and value-at-risk: Relative performance in emerging markets’, International Journal of Forecasting 20(2), 287–303. https://doi.org/10.1016/j.ijforecast.2003.09.005
Gilli, M. & Këllezi, E., 2006, ‘An application of extreme value theory for measuring financial risk’, Computational Economics 27(2), 207–228. https://doi.org/10.1007/s10614-006-9025-7
Katz, R.W., Parlange, M.B. & Naveau, P., 2002, ‘Statistics of extremes in hydrology’, Advances in Water Resources 25, 1287–1304. https://doi.org/10.1016/S0309-1708(02)00056-8
Kiragu, K.A. & Mung’atu, J.K., 2016, ‘Extreme values modelling of Nairobi securities exchange index’, American Journal of Theoretical and Applied Statistics 5(4), 234–241. https://doi.org/10.11648/j.ajtas.20160504.20
Longin, F.M., 2000, ‘From value at risk to stress testing, the extreme value approach’, Journal of Baking and Finance 24(7), 1097–1130. https://doi.org/10.1016/S0378-4266(99)00077-1
Loretan, M. & Phillips, P., 1994, ‘Testing the covariance stationarity of heavy-tailed time series’, Journal of Empirical Finance 1(2), 211–248. https://doi.org/10.1016/0927-5398(94)90004-3
Nortey, N.N., Asare, K. & Mettle, F.O., 2015, ‘Extreme value modelling of the Ghana Stock Exchange Index’, Springerplus 4, 696. https://doi.org/10.1186/s40064-015-1306-y
Magnou, G., 2016, An application of extreme value theory for measuring financial risk in the Uruguayan Pension Fund 1, Department of Legal, Compliance and Risk, University of the Republic, Uruguay.
McNeil, A.J., 1997, ‘Estimating the tails of loss severity distributions using extreme value theory’, ASTIN Bulletin 27, 117–137. https://doi.org/10.2143/AST.27.1.563210
Omari, C.O., Mwita, P.N. & Waititu, A.G., 2017, ‘Using conditional extreme value theory to estimate value-at-risk for daily currency exchange rates’, Journal of Mathematical Finance 7, 846–870. https://doi.org/10.4236/jmf.2017.74045
Onour, I.A., 2010, ‘Extreme risk and fat-tails distribution model: Empirical analysis’, Journal of Money, Investment and Banking 13.
Pickands, J., 1975, ‘Statistical inference using extreme order statistics’, Annals of Statistics 3, 119–131. https://doi.org/10.1214/aos/1176343003
Ren, F. & Giles, D., 2010, ‘Extreme value analysis of daily Canadian crude oil prices’, Applied Financial Economics, Taylor & Francis Journals 20(12), 941–954. https://doi.org/10.1080/09603101003724323
Rydell, S., 2013, ‘The use of extreme value theory and time series analysis to estimate risk measures for extreme events’, Master’s thesis in Engineering Physics, Department of Physics, Umeå University.
South African Financial Index (J580) data, (1995–2018), iress expert, viewed 04 February 2018, from https://expert.inetbfa.com.
Vee, D.C.N., Gonpot, P.N. & Sookia, N., 2014, ‘An application of extreme value theory as a risk measurement approach in frontier markets world academy of science, engineering and technology’, International Journal of Economics and Management Engineering 8(6), 2014.
Vladimir, O., Sergey, E.T., Oksana, P.O. & Gennady, P., 2016, ‘Extreme value theory and peaks over threshold model in the Russian Stock Market’, Journal of Siberian Federal University. Engineering & Technologies 1(5), 111–121.
Zhaoa, X., Scarrotta, C., Oxleyb, L. & Realea, M., 2010, ‘Extreme value modelling for forecasting market crisis impacts’, Applied Financial Economics 20, 63–72. https://doi.org/10.1080/09603100903262947
|