Abstract
Background: Financial market participation is explained from the viewpoint of financial literacy, awareness, investors’ risk aversion, and cost of stock market participation but could not be successful. As a result, households’ lack of participation in the stock market remains a puzzle across the world.
Aim: The paper examines the factors determining the participation of individuals in the financial markets using the logistic regression model. Categorical data covering a sample of 484 were collected from individuals residing in Arusha, Tanzania.
Conclusion: Male and married individuals, as well as people with financial knowledge have a better chance of investing in the financial market despite their education level. Other variables such as risk attitude and level of income play a significant role in influencing individuals’ participation in the financial markets. The policy implication of these results is that increasing training, awareness of the benefits and operations of the financial markets will result in people opting to participate in the financial market, which will, in turn, lead to increased trading of financial assets and hence create a ripple effect to the economy.
Keywords: financial literacy; financial markets; logit modelling; regression model; Tanzania.
Introduction
Limited level of financial market participation is a long-standing puzzle in empirical finance. The phenomenon is observed in many countries across the world and indeed, participation rates vary widely even amongst developed countries. Many studies explain the phenomenon from the viewpoint of financial literacy, awareness, investors’ risk aversion and cost of stock market participation but could not be successful (Almenberg & Dreber 2015; Arts 2018; Dillen & De Lille 2018; Gumbo & Sandada 2018; Sindambiwe 2014; Van Rooij, Lusardi & Alessie 2011). As a result, households’ lack of participation in the stock market remains a puzzle across the world (Kadoya, Khan & Rabbani 2017).
The Bank of Tanzania Act of 1995 paved way for development of financial markets in the country. The main purpose was to promote and facilitate the development of an orderly, fair and efficient capital market and securities in Tanzania. According to the Bank of Tanzania (2018), financial markets in Tanzania consist of money, bonds, equities, foreign exchanges and collective investment schemes. Also, according to the Financial Inclusion Report (2017), 56% of adults in Tanzania are financially included through the use of mobile banking services. However, despite the fact that in 2006 the Banking and Financial Institutions’ Act was formed to ensure that there are stable, safe and sound financial systems; banks and pension funds are preeminent in Tanzania’s small financial sector (IMF 2018). Financial sector assets are 36% of Gross Domestic Product (GDP), with banks (72% of system assets) and pension funds (26% of system assets) being systemic components. In Kenya, for example, the share of financial sector assets in GDP is 76% (Financial Sector Regulators 2019). More significantly, the financial market in Tanzania fails to reach its objectives because of a number of challenges including low financial education and literacy as well as low access to financial services to the majority of Tanzanians (Macha 2009). Although Tanzania decided to embark on financial liberalisation in 1991 to sustain its growth, as it considers the capital market development an important component of the economic recovery programme, its financial market is still described as an emerging market with only 28 companies listed in Dar es Salaam Stock Exchange (DSE) with a total market capitalisation of Tanzanian shillings (TZS) 20186.48 billion, equivalent to US$ 8.7 billion, as of 18 December 2015. Out of 44.9 million Tanzanian citizens (NBS 2012), 52% are estimated to be working-class in which only 200 000 are individual investors participating in the financial markets in Tanzania. This approximates 0.9% of total working-class participants.
Understandably, as Babu (2018), Shahabadi and Jafari (2017) and Ziorklui (2001) argue, financial market institutions such as the stock market can assist better in the mobilisation of domestic capital. This is because of the fact that they offer a simple mechanism for fund transfer which encourages investment. They also permit companies to have access to a larger number of investors in comparison to private-owned companies. Owing to this, the growth and development of the financial markets are of vital importance to the country as well as individuals’ economy. However, for any market to progress and people to participate effectively there is a need for awareness and education on how the market operates (Balloch, Nicolae & Philip 2015). Financial decision making is affected by an individual’s level of financial literacy since low literate individuals are less likely to invest in stocks and therefore are less likely to participate in the stock market (Arts 2018; Aubert, Kammoun & Bekrar 2018; Van Rooij et al. 2011).
Although many studies discuss the relevance of financial literacy to financial market participation and the importance of the latter, participation rates still are considerably low (Andersen & Nielsen 2011; Arts 2018; Van Rooij et al. 2011). Additionally, substantial differences exist in stock market participation rates between countries (Guiso, Haliassos & Jappelli 2003). Nonetheless, evidence regarding the impact of financial illiteracy on financial behavior has been both scarce and mixed (Agarwal et al. 2011; Martin 2007). One reason for these limitations is that a substantial fraction of existing studies that address this question are based on the evaluation of specific financial education programs and policies (Yoong 2011). Indeed, according to Bayer, Bernheim and Scholz (1996) and Bernheim, Garrett and Maki (2001), employer-based financial education increases participation in saving plans, whilst financial education significantly increases the adult propensity to save. However, other studies such as Cole and Shastry (2009) and Duflo and Saez (2003) have found small impacts of financial education on financial decision-making. Notably, financial education programmes may fail to affect literacy (Yoong 2011). By contrast, Hilgert, Hogarth and Beverly (2003) find that individuals with more financial knowledge are more likely to engage in recommended financial practices. Similarly, Lusardi and Mitchell (2006, 2007) demonstrate that individuals with better financial knowledge are more likely to plan and to invest in complex assets.
The relationship between financial literacy and participation in the financial market is generally complex. Studies (e.g. Almenberg & Dreber 2015; Georgarakos & Pasini 2011; Hong, Kubik & Stein 2004) show that financial literacy and education apart, other individuals’ characteristics namely, age, gender, wealth and risk aversion, may influence financial market participation. Nevertheless, like financial literacy, the effect of other individuals’ characteristics on financial market participation is controversial. For example, according to Georgarakos and Pasini (2011), households with a similar level of average wealth holdings are likely to have different rates of financial market participation rates. They differ even to such extent that households with lower average net wealth holdings have higher financial market participation rates than households with higher average net wealth holdings (Georgarakos & Pasini 2011). This revelation indicates that more investigations and analyses of the effect of financial literacy, awareness and other individual characteristics on participation in the financial market are of paramount importance.
This article, therefore, empirically examines the effect of financial literacy and other factors, namely age group, marital status, level of education, level of income, risk attitude and self-assessed financial knowledge on participation in the financial markets in Tanzania, taking a case study of Arusha city, using categorical data and logistic regression analysis. The article is confined itself to DSE market and mutual fund market: Unit Trust of Tanzania (UTT), with the aim of providing policy recommendations that help to improve marketing strategy and individual financial market participation and investment. The rationale behind investigating the relationship between financial literacy and other individual characteristics on the financial market participation is that previous studies show mixed findings. More significantly so, the improvement of public awareness about financial market investment would increase the rate of participation, which would in turn lead to economic growth and development. This is very important because financial markets play an important role in the economy by creating the open and regulatory system for the firms to acquire amounts of capital resulting in companies issuing shares to the public. This helps the companies to raise enough capital for businesses through selling company shares and bonds. The important benefit of this is reflected in the individual’s savings along with investments in the securities which then promote commerce and the industry as opposed to idle bank deposits (Antwi-Asare & Addison 2000; Fohlin 1999; Saeed 1971).
Literature review
The degree of involvement in the financial markets is greatly influenced by the awareness and knowledge of such market’s operations, risks and benefits involved (Andersen & Nielsen 2011; Arts 2018; Lusardi & Mitchell 2007a, 2014; Van Rooij et al. 2011). Several studies have attempted to find the degree of association between participation, awareness and literacy in the financial market. For example, Guiso and Japelli (2005) find that lack of awareness as well as ignorance on the investment opportunities are one of the major reasons that many people do not invest in mutual funds, investment accounts, stocks and corporate bonds. The implication is that if all investors are aware of the risk involved in the participation of the investment activities, then many will invest and thus there will be an increase in the investment activities. In addition, if consumers are greatly aware of the available financial assets then they will be more willing to participate in the markets. Indeed, Guiso and Japelli’s (2005) findings suggest that forms of information dissemination such as training, advertisement and incentives may make individuals aware of financial markets.
As has been stated, the relationship between individual characteristics and participation in the financial markets is inclusive. According to Sindambiwe (2014), individuals in managerial positions are more skilled and aware of the operations of the financial markets. They measure high their financial literacy in terms of investment in regards to interest rate calculations, exchange rate calculations, business diversifications, portfolio management and stock market location, but they have little awareness of the existence of brokerage services (Sindambiwe 2014). Notably, despite the knowledge of the financial market, the level of participation in the financial market is low mainly because companies do not have important information and an opportunity for investing, mainly because of a lack of financial advisors (Sindambiwe 2014). This, however, suggests that education and other factors such as demographics and level of income play a role in the decision to participate in the financial market. For example, education is likely to influence the level of awareness and participation in which those with higher education are observed to be more aware compared to the ones with lesser education. Also, Guiso and Japelli (2005) demonstrate that individuals with a surplus, based on the household income, are more likely to invest compared to those without or with a lesser surplus. Indeed, individuals with a higher level of income have a higher literacy level compared to those with a lower level of income (Klapper, Lusardi & Van Oudheusden 2015). However, according to Sindambiwe (2014), knowledge of the financial market is a major factor in comparison to demographics and income level because it leads to the belief in the returns obtained as a result of investing in the markets. In a similar study, Gumbo and Sandada (2018) argue that education is not the main factor influencing financial market participation. Indeed, Gumbo and Sandada (2018) show that individuals with higher education levels participate less in comparison to individuals with lower education levels. According to Gumbo and Sandada (2018), awareness, cognitive skills and transaction costs are a significant factor in participation in the financial market. Similarly, Omole and Falokun (1999) reveal that a few firms participate in the financial market because individuals’ awareness of financial markets is restricted in the Nigerian stock exchange. The government favours the money market rather than the capital markets (Omole & Falokun 1999). In Tanzania, the effort that is made through the media by DSE and UTT is still not enough and therefore, there is a need for further investigation and hence proper recommendations (Massele et al. 2013). Despite the fact that many studies show a lack of awareness is likely to affect participation in the financial markets, there is still a requirement for further investigation on what level of awareness is required and if at all, this is a major factor of influence in the selection of investing in Tanzania.
Financial literacy could range from fairly knowledgeable to highly knowledgeable of the undertakings of the financial markets. It is not a necessity to be very knowledgeable of the financial markets and operations, and some studies show that just a basic knowledge can cause individuals to participate in the financial markets (Van Rooij et al. 2011). For one to be financially literate, they should be able to understand the concepts of financial workings including workings of interest rate, compounding interest, inflation and risk diversification (Klapper et al. 2015). It is worth noting that education is not a good proxy for financial literacy. That is, according to Lusardi and Mitchell (2011), when education and financial literacy are included in multivariate regression models of financial market participation, both tend to be statistically significant, indicating that financial literacy has an effect above and beyond education. García and Tessada (2013) analyse the influence of education on the participation in the financial markets. Their findings show that the increase in the level of education increases the probability of participation by 3%. They conclude that education has a major influence on the individual’s participation in the financial markets.
Financial illiteracy is widespread even in well-developed financial markets as in Germany, the Netherlands, Sweden, Italy, Japan and New Zealand (Lusardi & Mitchell 2011). Thus, observed low levels of financial literacy in developing countries such as Tanzania are prevalent elsewhere, rather than being specific to any given country or stage of economic development. Furthermore, financial literacy rates differ in important ways when it comes to characteristics such as gender, education level, income and age. Previous studies show that men are more financially literate than women (Lusardi & Mitchell 2014). Worldwide, 35% of men are financially literate, compared to 30% of women (Klapper et al. 2015). Women have poor financial skills than men even considering variations in age, country, education and income (Klapper et al. 2015). According to Klapper et al. (2015), there is a slight variation in the average gender gap in financial literacy between developed and emerging markets. Although women tend to be better decision-makers when it comes to money management and investment, the limited knowledge of the stock market plays a big factor in lacking investment thereof (Kulkarni & Deepa 2019). The psychological and cultural norms are also at play when gender is concerned, with the notion that males dominate the economy and investment decisions. Despite the fact that females comprise 40% of the overall workforce, they are most unlikely to be educated on financial skills (Isaac 2014).
Moreover, for the emerging markets, youth are more informed and knowledgeable in financial education compared to those above 35 years of age (Klapper et al. 2015). However, this is contrary to a developing economy where individuals above 35 years of age are more financially literate than the youth (Klapper et al. 2015).
Studies on people’s participation in the financial markets show that trust in reference to the financial market is how a person can perceive the involved risk and benefits, and decide that the perceived benefits outweigh the risk and hence safe for the individual’s investment (see e.g. Balloch et al. 2015). Balloch et al. (2015) reveal that stock market literacy and trust in the stock markets became the resulting factors for the households to invest in the stock markets. Different individuals have different perceptions of risk. The knowledge of the financial market place may guarantee even the risk-averse individual a position to take the risk. Cheng et al. (2018) argue that if a household can identify and understand the stocks better, they can choose the amount of risk they are willing to sustain. According to Yao, Hanna and Montalto (2003), despite the fact that females have higher life expectancy, they are keener and risk-averse. Males are more prone to taking higher risks. Also, as mentioned earlier, age seems to be a factor of interest in the participation of the financial markets. Financial Engines (2018) demonstrate that older people are more careful considering they have greater experience and have picked up skills along the way. In addition, as individuals’ age increase, they are more likely to accumulate wealth and hence engage in the financial market investments (Kartik, Ottaviani & Squintani 2016).
The financial market participation puzzle is relatively incomplete. Despite its proven importance, previous studies still could not completely rationalise the rather low financial market participation rates and the factors determining market participation rates. By and large previous studies, for example, Cheng et al. (2018), García and Tessada (2013), Gumbo and Sandada (2018) and Sindambiwe (2014) examine the factors determining participation in the financial markets, specifically stock markets. They focus more on the households and organisations whilst identifying the factors that influence participation in the stock markets and capital markets. Factors such as age, education level, gender, risk attitude, marital status and self-assessed financial knowledge are widely used in previous studies. It is worth noting however, that individual participation in the financial markets in Tanzania has been rarely analysed and hence needs more investigation. Theories indicate that individual awareness in the financial market and consequential participation in the financial markets is of great importance, and therefore there is a need for empirical justification in a country such as Tanzania, in which its financial market is still developing. Overall, this article focuses mainly on the factors influencing the individual investor’s decisions to participate in the financial markets taking multiple variables, namely age group, gender, marital status, education level, risk attitude, income level and self-assessment of the financial literacy into account.
Conceptual framework
This article analyses financial literacy and individuals’ participation in the financial markets, based on the conceptual framework reported in Figure 1. This framework presents the key variables that are analysed using the logistic regression model and maximum likelihood (ML) estimation technique as discussed in the ‘Methodology’ section.
All regressors and regressand are categorical variables and their measurements are presented and discussed in detail in the ‘Methodology’ section. The causal relationship between individual regressors and regressand can be explained as follows: firstly, as far as the level of education is concerned, several studies have revealed that a person with a higher level of education is equipped with better financial knowledge (Sindambiwe 2014). Secondly, as previous studies have revealed, women are more risk-averse and also good planners (Kulkarni & Deepa 2019; Yao et al. 2003). Hence, gender plays a significant role in financial investment. Thirdly, it is believed that the more people have to spare as surplus income, the more they will be willing to invest in different instruments including financial sectors (Cheng et al. 2018; Guiso & Japelli 2005). Thus, individuals with a higher level of income are more likely to participate in the financial market. Fourthly, age plays a key role in risk-taking. Studies have shown that the younger the person, the more willing they are to take riskier options (Kartik et al. 2016). For this reason, the age-group plays a significant role in determining whether a person can or is willing to invest in several financial instruments. Fifthly, researchers have found that a married individual is less likely to invest in riskier markets and make the riskier financial decisions in comparison to the single individual. Thus, it is important to analyse if marital status influences the participation of the financial markets. Sixthly, there are three categories of an individual’s attitudes to risk, namely risk-averse, risk-takers and risk-neutral. The risk attitude can majorly influence one to invest in the financial market if all factors are left constant (Balloch et al. 2015). Therefore, risk attitude is one of the factors examined to establish if an individual can participate in financial markets based on riskiness. As shown in the ‘Methodology’ section, the risk attitude is being given a score so as to identify the risk character of the participants. Lastly, the article assesses the perceived financial literacy of the individual to see how individuals evaluate their financial knowledge and participation in the financial market. To assess financial literacy, individuals state how they think they know their level of knowledge in relation to finance by rating their knowledge between scales of 1 and 10. This also is discussed in the ‘Methodology’ section. In summary, the coefficients of the level of education, gender, level of income, age group, marital status and self-assessed financial knowledge are expected to be positive whereas the coefficient of risk attitude is expected to be negative.
Methodology
Model specification
The logit regression model is adopted appropriately to examine a cause–effect relationship between the variables. Logit or logistic regression analysis studies the relationship between a categorical dependent variable and a set of independent variables. The logistic regression is truly applicable here since the dependent variable has only two values, that is 0 and 1 or Yes and No. Logit regression is a nonlinear regression model designed for binary dependent variables. Because regressions with a binary dependent y model the probability y = 1, it makes sense to adopt a nonlinear formulation that forces the predicted value to be between zero and one (Stock & Watson 2007). Indeed, the logit model is a significant improvement on the linear probability model. It is based on the cumulative distribution function (CDF) of a random variable, with the logit model following the logistic CDF, giving the relationship as shown in Figure 2 (GraphPad 2009). As shown in Figure 2, the regression line is nonlinear, giving a more realistic description of the data, with very little change in the probability at the extreme value that the explanatory variable can take. As has been stated, in the logit model, the probability of the dependent variable takes the value of 1 for a given value of the explanatory variable. This method is better suited for modelling and analysing categorical-response variables because it does not assume that the independent variables are normally distributed.
Consider a linear regression model (Eqn 1) and associated probability function (Eqn 2). Where y is continuous whilst the xs can be purely continuous, dichotomous (dummy variables) or both. Recall that a continuous variable is one that can take any value between two numbers:
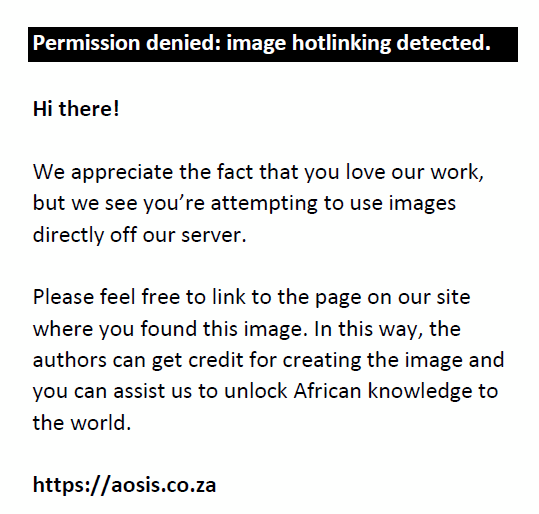
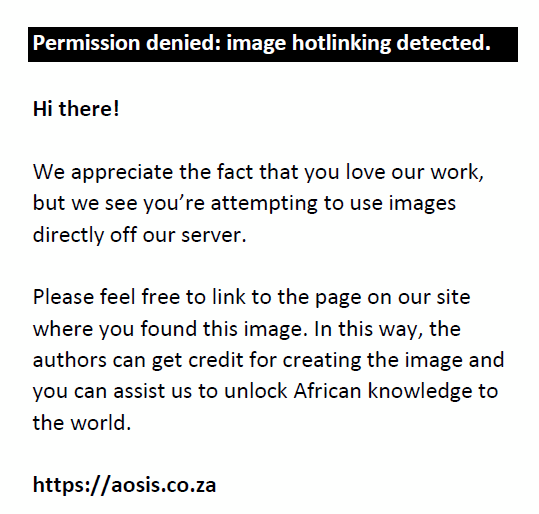
where pi is the probability of financial market participation; x1 is the gender; x2 is the age group; x3 is the marital status; x4 is the education level; x5 is the monthly income; x6 is the risk assessment; x7 is the self-assessed financial knowledge; β0, β1, β2,…,β7 are the parameters and ɛ1 is the disturbance term.
The cumulative logistic distributive function can then be written as:
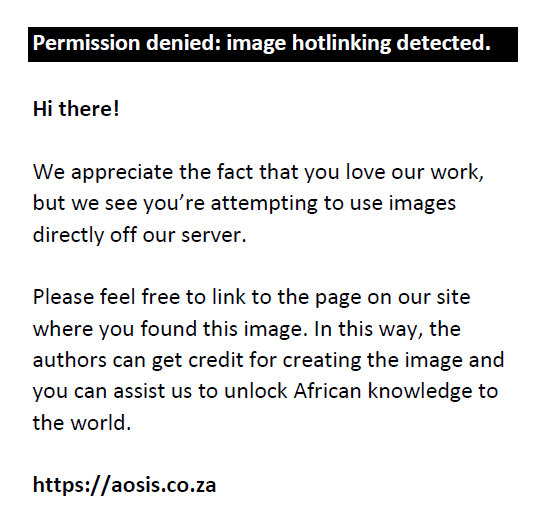
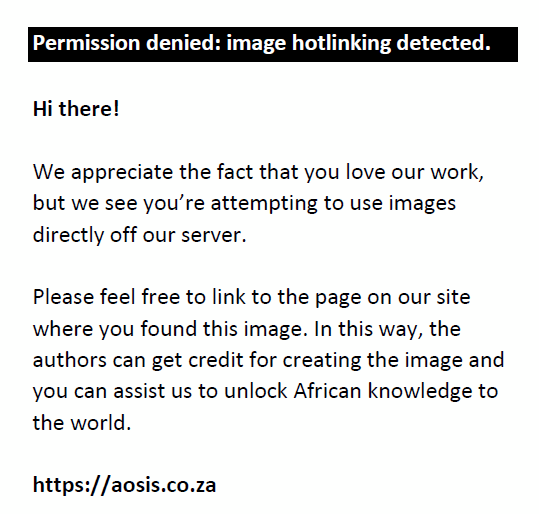
where the probability of financial market participation pi is equal to 1. e is the exponential under the logit approach. Then, the logistic model estimated would be specified as:

The model is then expressed as the odds ratio, which is simply the probability of financial market participation relative to the probability of nonparticipation in the financial market. Given that:
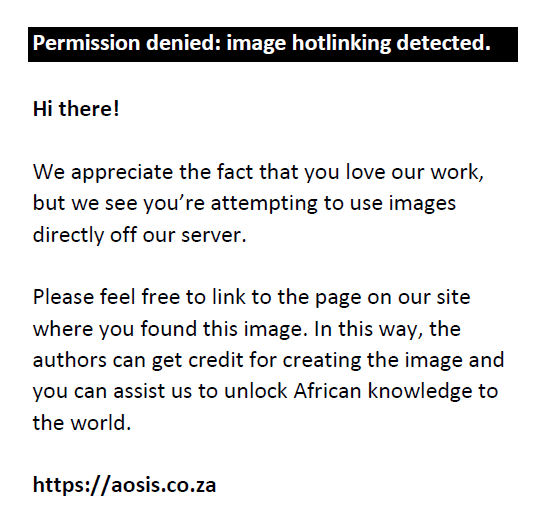
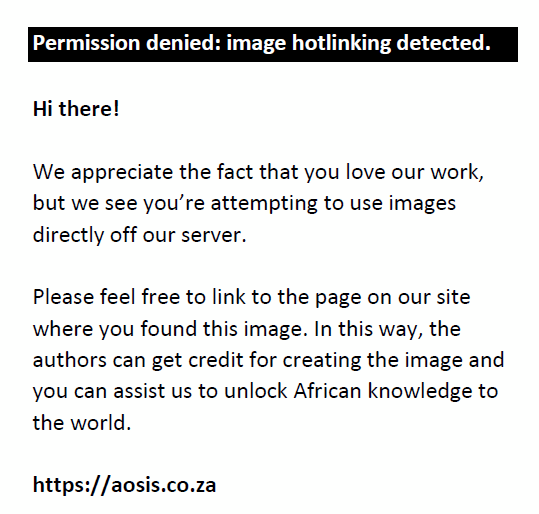
The ratio Pi/(1-pi) is the odds ratio of financial market participation. For example, if the probability of financial market participation pi is 0.75, then the probability of nonparticipation in the financial market 1-pi is 0.25. The odds of participation in the financial market are defined as the ratio of the probability of financial market participation over the probability of nonparticipation in the financial market. Thus, the odds of financial market participants will be 0.75/0.25 = 3 This will imply that the odds of financial market participants are 3 to 1. Then by taking the natural logarithm of the odds ratio, we produce the logit (Li), as follows:
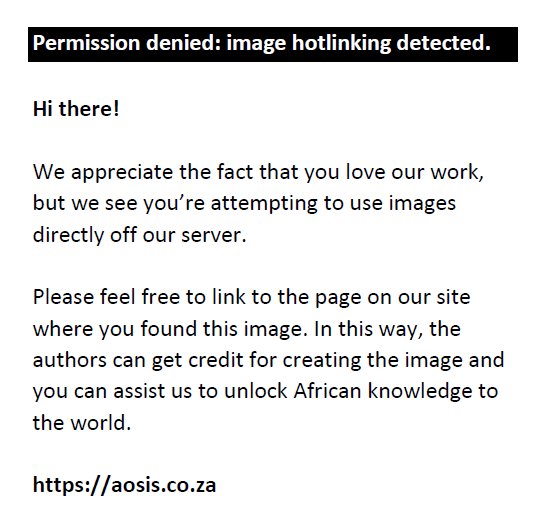
Model (Eqn 8) suggests that L is linear in x; the probabilities (p) are not linear. The logit model can be used in multiple regression tests. The slope coefficient measures the change in the log-odds ratio for a unit change in the explanatory variable. Once the logit has been estimated, hypothesis testing and econometric analysis can be undertaken in much the same way as for linear equations. If L is positive, as the value of the explanatory variables increases, the odds that the dependent variable equals 1 increase. The model estimates for each respondent the logarithm of the probability of financial market participants to the probability of not participating in the financial market. Table 1 presents the definitions, description and measurements of key variables that are included in the logit model.
TABLE 1: Definitions and measurement of key variables. |
Sample size, nature of data and data collection method
The article targeted the adult population within Arusha city who were randomly selected. The adult population was selected because of the likelihood to have been heard of or participated in the market and hence would be able to provide viable feedback. The article therefore, targeted 130 000 individuals in Arusha city (NBS 2012). The sample size was computed using Yamane (1967) probability sampling technique. In common practice, Yamane (1967) provides a simplified formula to calculate the sample size. This simplified population proportion method that corrects the weaknesses found in Cochran and the finite population correction methods, and assuming a 95% confidence level and ρ = 0.5 can be expressed as follows:
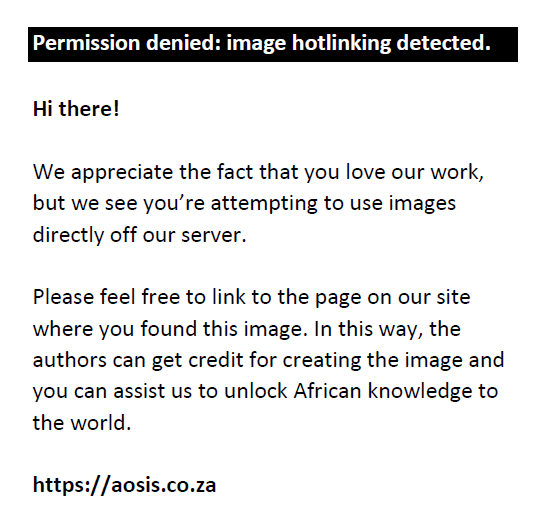
where n is the sample size; N is the population size and e is the acceptable sampling error or the error precision level.
Applying expression (Eqn 9), the sample size (n) of 130 000 population (N) was obtained as follows:
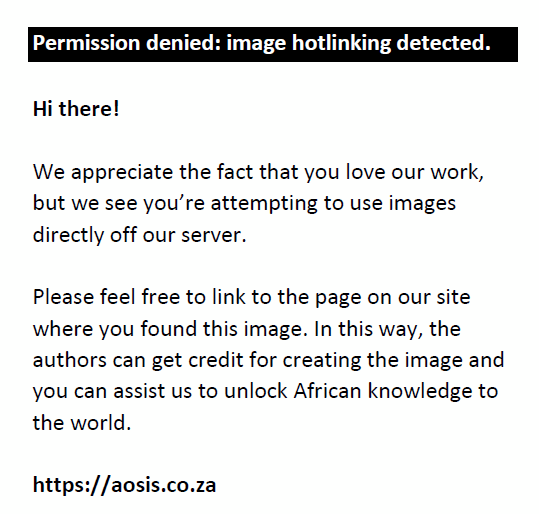
However, the fact that the logistic model requires a big sample size, the study managed to obtain 484 respondents; hence a sample size of 484 individuals was used for analysis. Indeed, the sample size of 484 is appropriate for logistic regression (Hsieh 1989).
Data were collected using the online questionnaire. Questionnaires were distributed through the Internet and once complete were returned via email. Questions were randomly administered to several people selected randomly given a period of 2 weeks to respond and return the questionnaires. This approach could quickly and easily capture the relevant information from a large number of respondents in a short period of time. The questionnaire captured relevant information such as the general profile of the respondent including location, age, gender, income, marital status as well as education level. It also captured significant information such as risk assessment, financial literacy assessment, as well as financial market participation of the respondent.
To ensure that there is no bias in the data, the randomisation technique was used. This technique helps to eliminate selection and confounding bias, thus guaranteeing the groups are comparable regardless of the occurrence of factors other than those being examined (Kang 2013). A pilot test was administered to ensure the applicability and suitability of the content, clarity and sufficiency of the instruments, which would in turn ensure the validity and reliability of data. Equally important to ensure that data are reliable and that they can be used in testing other models, Cronbach’s alpha test was applied. The Cronbach’s alpha test measures the internal consistency of data; it is the measure of scale reliability (Gliem & Gliem 2003). It was computed by correlating score for each question in the questionnaire and results are reported in Table 2. Since the scale reliability coefficient is 0.7, a value between 0.65 and 0.8, data have internal reliability. Usually, the coefficients below 0.5 are unacceptable. Equally noteworthy, the chi-square goodness of fit test is used to check for the consistency of random distribution of the participants. Results are reported in Table 3. Since the probability of χ2 = 0.009 is less than the 0.05 level of significance, we fail to reject the null hypothesis that data are consistent with random distribution.
TABLE 2: Cronbach’s alpha tests for reliability. |
TABLE 3: Chi-square goodness-of-fit test. |
Table 4 provides a summary of the categorical responses of all 484 individuals who participated in the process of data collection. Qualitative data are based on the number or percentage of financial market participation. Responses also are itemised into gender, age group and marital status. Other categories of respondents include education level, monthly income, self-assessed financial knowledge and attitude towards risk. Presumably, since financial markets rise and fall at any given time, resulting in gains and losses, individuals more inclined to take risks are more willing to invest in financial trading. As reported in Table 4, 69.6% of the respondents have a positive risk attitude. It is also revealed that about 93.2% of the respondents seem to have either fair, or moderate, or advanced knowledge of the financial market. Surprisingly, however, only 17% of the respondents with a diploma or degree level of education participate in the financial market whilst 59% of respondents with primary or informal education participate in the financial market.
TABLE 4: Descriptive analysis of the variables. |
Estimation method: Maximum likelihood
As has been presented, logistic regression is commonly applied to model the outcomes of a categorical dependent variable. It is inappropriate to use linear regression for categorical variables because the response values are not measured on a ratio scale and the error terms are not normally distributed. In addition, a categorical variable such as participation in the financial market can only take on a limited number of discrete values within a specified range whereas the linear regression model can be generated as predicted values of any real number ranging from negative infinity to positive infinity.
Logistic regression predicts probabilities, thus one can fit it using likelihood. For each data-point, there is a vector of features xi and an observed class yi. The probability of that class, as discussed above, is p, if yi = 1 or (1-p) if yi = 0. The likelihood function for logistic regression is expressed as:
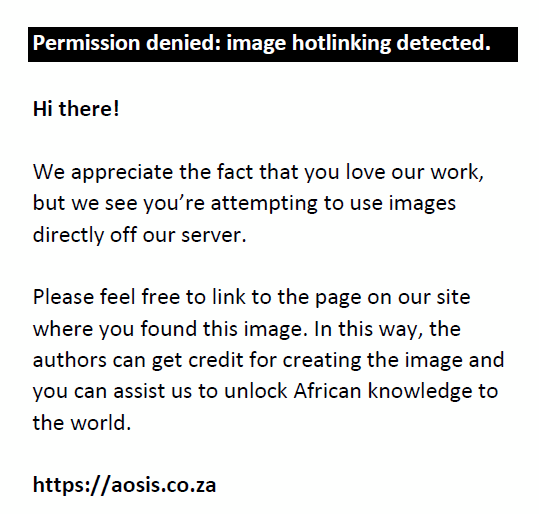
Then, the log-likelihood function is given as:
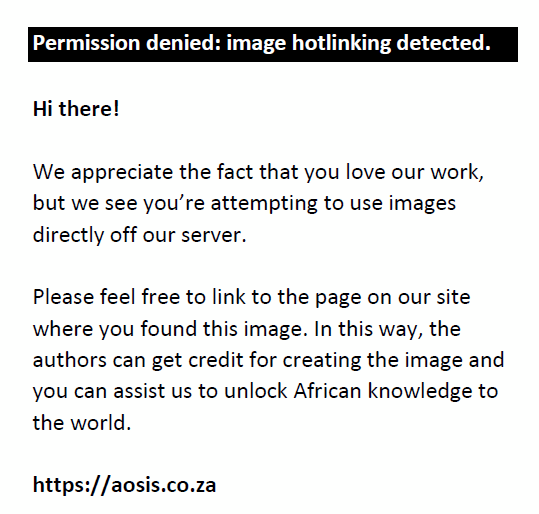
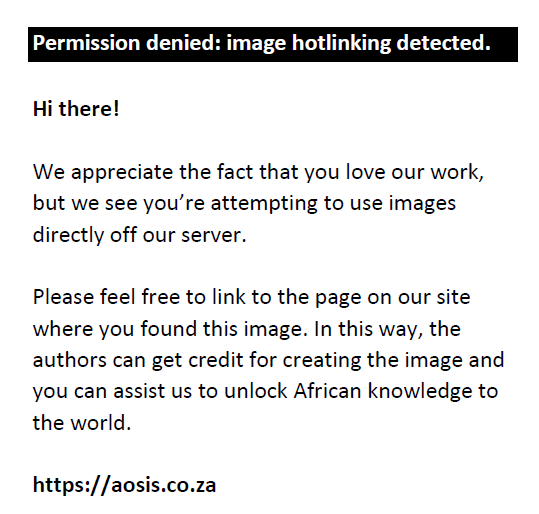
To find the ML estimates, we differentiate the log-likelihood with respect to the parameters and set the derivatives equal to zero to get the estimates. Since this equation is nonlinear in β, some special methods should be used in order to obtain the estimated parameters. The iteratively re-weighted least squares (IRLS) method can be applied to get the solutions. The ML estimator of β can be obtained by using the IRLS algorithm as follows:
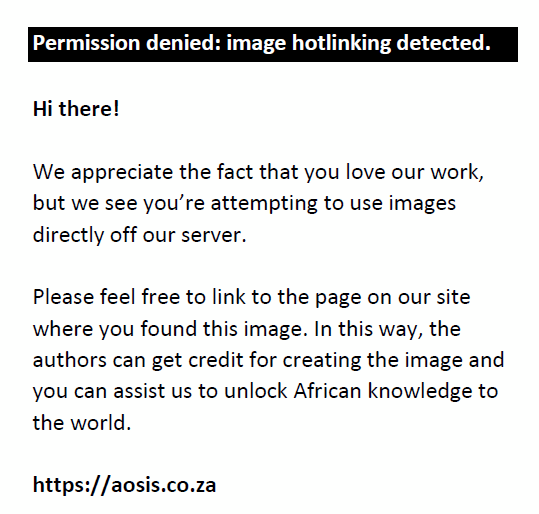
This is the ith element of the vector Ẑ. The hats in the equations show the iterative process.
Empirical results
Table 5 presents the results of the logit regression using Stata. The regressand is the probability of financial market participation (participate), whereas regressors are gender, age group (age), marital status (marital), level of education (education), monthly income (income), risk assessment (risk) and self-assessed financial knowledge (knowledge). It should be noted that when a binary outcome variable is modelled using logistic regression, it is assumed that the logit transformation of the outcome variable has a linear relationship with the predictor variables. The Log likelihood is the logarithmic transformation of the likelihood function of the final model. The value −234.8114 has no meaning in and of itself; rather, this number can be used to help compare nested models. The number of ‘obs’ is the number of observations that is used in the analysis. Since Stata uses a list-wise deletion by default, if there is a missing value for any variable in the logistic regression, the entire case will be excluded from the analysis as a result this number may be smaller than the total number of observations in the data set.
LR chi2(7) is the likelihood ratio (LR) chi-square test. This is minus two (i.e. −2) times the difference between the starting and ending log likelihood. The number in the parenthesis indicates the number of degrees of freedom. In this model, there are seven predictors, so there are seven degrees of freedom. Prob > chi2 is the probability of obtaining the chi-square statistic given that the null hypothesis is true. In other words, this is the probability of obtaining the chi-square statistic 99.53, if there is in fact no joint effect of the regressors on the regressand. It is the p-value, which is compared to a critical value, say 0.05 or 0.01 to determine if the overall model is statistically significant. In this case, the model is statistically significant because the p-value is 0.000. Pseudo R2 is the pseudo R-squared. It should be noted that this statistic does not mean what R-square means in Ordinary Least Squares (OLS) regression.
The constant, in this model, is the expected value of the log-odds of financial market participation when all of the independent variables equal zero. In most cases, this is not interesting. Similar to linear regression, the standard error associated with the coefficient (Std. Err.) is used for testing whether the parameter is significantly different from zero. The standard errors can also be used to form a confidence interval for the parameter. z and P > |z| provide the z-value and two-tailed p-value used in testing the null hypothesis that the coefficient (parameter) is 0. Coefficients having a p-value of 0.05 or less are statistically significant. That is, we can reject the null hypothesis and say that the coefficient is significantly different from zero. The (95% Conf. Interval) shows a 95% confidence interval for the coefficient. This is very useful as it helps us understand how high and how low the actual population value of the parameter might be. The confidence intervals are related to the p-values such that the coefficient will not be statistically significant if the confidence interval includes 0.
The estimates:
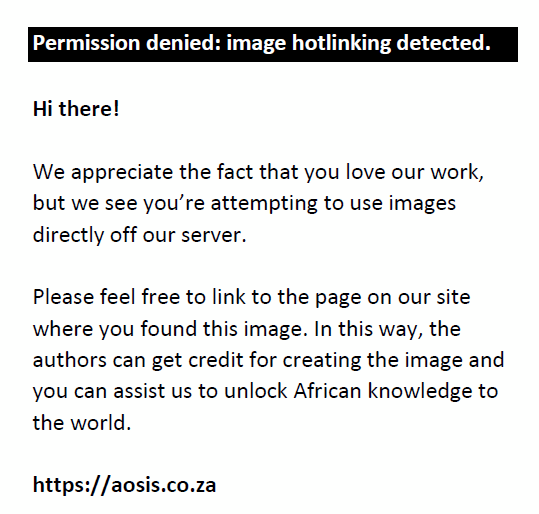
where the coefficients and variables are described in Table 5, tell us about the relationship between the independent variables and the dependent variable, where the dependent variable is on the logit scale. These estimates show the amount of increase in the predicted log odds of financial market participation = 1 that would be predicted by a 1 unit increase in the predictor, holding all other predictors constant. It is worth noting that for the regressors which are not significant, the coefficients are not significantly different from zero, which should be taken into account when interpreting the coefficients. Table 6 reports the decisions on the significance of individual coefficients. Because these regression coefficients are in log-odds units, their interpretation is somewhat tricky, so they are often converted into odds ratios. This means that the coefficients in a simple logistic regression are in terms of the log odds, that is, the coefficient of gender of 0.6189526 implies that a one unit change in gender results in a 0.6189526 unit change in the log of the odds. Odds ratios are obtained by exponentiating the coefficient, or by using the logistic command. Odds ratio results are reported in Table 7.
TABLE 6: Decisions on the significance of individual coefficients. |
TABLE 7: Logistic regression: Odds ratio. |
Odds of financial market participation are about 1.86 greater for males than females whilst the odds of financial market participation for the age group of 25+ years and married individuals are 1.36 and 2.92 times that of the age group below 25 years and single/divorced participants, respectively. Likewise, the odds of financial market participation for individuals with monthly income above TZS 300 000 and individuals with fair, or moderate, or advanced knowledge of the financial market are 1.67 and 2.85 times that of individuals with monthly income below TZS 300 000, and individuals with poor knowledge of financial knowledge, respectively. Moreover, the odds of participating in the financial market for risk-taker are about 1.54 times that of risk-averse. Surprisingly, the odds of participating in the financial market for individuals with education level above primary are 0.27 times that of individuals with primary or informal education. It should be noted that the odds ratio can vary between zero to positive infinity whilst the log odds ratio varies between (−∞ and ∞). Specifically, when the odds ratio lies between (0 and 1), the log odds ratio is negative.
For more intuition, it is also possible to compute the marginal effect of an independent variable on the probability. With binary independent variables, marginal effects measure discrete change, that is, how do predicted probabilities change as the binary independent variable changes from zero to one? The marginal effect of categorical variables, therefore, shows how P(Y = 1) changes as the categorical variable changes from zero to one, holding all other variables at their mean value. Results for marginal effects are reported in Table 8. In this case, the marginal effect for gender of 0.10 suggests that the predicted probability of financial market participation is 0.10 greater for male individuals than for female individuals, whilst marginal effects for age and marital status of 0.05 and 0.17 imply that the predicted probability of financial market participation is 0.05 and 0.17 greater for the age group of 25+ years and married individuals than for the age group below 25 years and single or divorced individuals, respectively. Marginal effect also suggests that the predicted probability of financial market participation is 1 lower for individuals with a level of education above primary, whilst the predicted probability of financial market participation for individuals with monthly income above TZS 300 000 is 0.08 greater than individuals with monthly income below TZS 300 000. Furthermore, the marginal effects show that the predicted probability of financial market participation for risk-takers and individuals with financial market knowledge are 0.07 and 0.17 higher than for risk-averse and individual with no knowledge of the financial market, respectively.
TABLE 8: Logistic regression: Marginal effects. |
In general, our empirical findings show that the coefficients on financial knowledge, gender, marital status and the level of education are statistically significant at 1% whilst the coefficients on income level and risk-taking are statistically significant at 10%. The coefficient on age group is insignificant suggesting that in our data sample age does not play a role in predicting financial market participation of individuals.
It was hypothesised that literacy of the financial market would positively influence the participation of individuals in the financial market. It is worth noting that financial literacy characterises an individual’s knowledge of basic concepts and it has been identified in this article to explain the variation in an individual’s tendency to participate in the financial markets. These findings suggest that individuals who are financially literate have the capacity to make more informed choices regarding financial matters, such as whether and how much to save. These results are consistent with many previous studies including Gerhard, Gladstone and Hoffmann (2018), Klapper et al. (2015), Lusardi et al. (2007a), Stango and Zinman (2009), and Van Rooij et al. (2011). Indeed, the largest effects in terms of the magnitudes of the marginal effects, reported in Table 8, come from the financial knowledge.
Similarly, it was presumed that the level of education would have a positive effect on the participation of individuals in the financial market. In fact, the coefficient of the level of education is significant; however, the effect is counter-intuitive and not consistent with some other empirical findings (e.g. Campbell 2006; García & Tessada 2013; Van Rooij et al. 2011), but consistent with other studies (e.g. Gumbo & Sandada 2018). Consistent with the findings of this article, Gumbo and Sandada (2018) reveal that individuals with higher education levels participate less in comparison to individuals with lower education levels. These results suggest that education is not the main factor influencing financial market participation. It should be noted that recent work on the impact of financial education on downstream financial behaviour suggests that traditional forms of education training have only limited effectiveness and points to the need for providing just-in-time financial training through, for example, couching (Fernandes, Lynch & Netemeyer 2014). Likewise, results suggest that, consistent with Bayer et al. (1996) and Bernheim et al. (2001), employer-based financial education that increases individuals’ financial knowledge is likely to increase the participation rate in the financial market. Financial literacy is likely to reduce information costs to a level that makes the financial market more attractive to individuals.
Empirical results also show that an individual’s income plays a significant role in financial market participation. The positive impact of the level of income on financial market participation is consistent with theories. Theoretically, labour income allows households to plan their consumption and follow the traditional consumption-smoothing theory where households that have excess income have the tendency to save or invest in order to maintain their same level of consumption in the future. Intuitively, the higher the income of an individual, the more likely the individual is to participate in the stock market. Moreover, logit regressions show that gender and marital status explain the variations in individuals’ participation in the financial market. The positive coefficients on gender and marital status imply that if the individual is a man or married, the more likely the individual is to participate in the financial market consistent with Dillen and De Lille (2018) and Isaac (2014). This also suggests that women are more risk-averse compared to men. They are reluctant to participate in the stock market as stocks are considered risky assets. Finally, our logit model shows that risk-taking is positively correlated with financial market participation. This implicates that the more risk-taker an individual is, the more likely the individual is to participate in the stock market.
Conclusions
The limited level of participation in the financial markets by individuals and households in the real world conflicts with theoretical understanding about the benefits of financial markets. At the same time, this limited participation can have important effects on the behaviour of financial markets. Our findings reveal that lack of financial literacy prevents households from participating in the financial market, even for individuals whose wealth, education and financial literacy are high relative to the general population. Financial knowledge, as a measure of financial literacy, is positive and highly significant, suggesting that financial literacy has a positive effect on individuals’ and households’ participation in the financial market. Indeed, the role of financial literacy in financial market participation cannot be overstated because the loss of welfare from nonparticipation in the financial market may be substantial. As individuals decide how much to save for future consumption and how to invest their income, it is important to consider ways to enhance their level of financial knowledge or to guide them in their financial decisions.
Surprisingly, results reveal that individuals with a primary and informal education are more likely to participate in the financial markets than their counterparts with a secondary and tertiary education suggesting that the educational attainment does not increase sharply with financial literacy. Financial literacy rates and participation in the financial market differ in important ways when it comes to characteristics such as gender, marital status, level of income and risk-taking. Men, married individuals and individuals with higher levels of income are more likely to participate in the financial market than women, single/divorced and individuals with lower monthly income. Similarly, individuals with high risk-taking attitude are more likely to participate in the financial market. However, age seems to exert no statistical influence on financial market participation.
These results shed additional light on the debates over financial literacy and individual characteristics, and provide policymakers and practitioners with new evidence linking financial literacy to financial behaviour. From an economic viewpoint, the fact that financial literacy and financial market participation are positively correlated, and the lack of financial literacy acts as a barrier to wealth-maximising investment, which ultimately impairs economic development. Proper financial education, seminars and financial orientation in the workplace can improve financial literacy and awareness, which in turn, can improve investment in the financial market.
Several potential avenues are available for future research. Firstly, the model does not account for a number of variables such as social interaction, compound interest, inflation and the time value of money, which may be relevant factors determining the financial market participation of individuals and households. Secondly, the article applies the logit model and ML techniques of estimation. Further studies may apply a two-stage least squares (2SLS) specification. Thirdly, the study focuses on the general financial market without specifying the primary or secondary market and also the stock market or mutual funds. Further studies could assess any of these markets separately.
Acknowledgements
The authors thank all anonymous reviewers for comments that greatly improved the manuscript.
Competing interests
The authors declare that they have no financial or personal relationships that may have inappropriately influenced them in writing this review article.
Authors’ contributions
M.E. and B.P.K. contributed equally to this review article.
Ethical considerations
This article followed all ethical standards for research without direct contact with human or animal subjects.
Funding information
This research received no specific grant from any funding agency in the public, commercial or not-for-profit sectors.
Data availability
The authors confirm that the data supporting the findings of this study are available within the article.
Disclaimer
The views and opinions expressed in this article are those of the authors and do not necessarily reflect the official policy or position of any affiliated agency of the authors.
References
Agarwal, S., Amromin, G., Ben-David, I., Chomsisengphet, S. & Evanoff, D.D., 2011, ‘Financial counseling, financial literacy, and household decision-making’, in O.S. Mitchell & A. Lusardi (eds.), Financial literacy: Implications for retirement security and the financial marketplace, Oxford University Press, Oxford.
Almenberg, J. & Dreber, A., 2015, ‘Gender, stock market participation and financial literacy’, Economics Letters 137, 140–142. https://doi.org/10.1016/j.econlet.2015.10.009
Antwi-Asare, T.O. & Addison, E.K.Y., 2000, Financial sector reforms and Bank performance in Ghana, ODI Research Study, Overseas Development Institute, University of Ghana, London.
Arts, L., 2018, ‘Financial literacy and stock market participation: The moderating effect of country-specific social connectedness’, Master’s thesis, Department of Business Studies, Uppsala University.
Aubert, N., Kammoun, N. & Bekrar, Y., 2018, ‘Financial decisions of the financially literate’, Finance 39(2), 43–91. https://doi.org/10.3917/fina.392.0043
Babu, G.S., 2018, ‘Role of financial system in economic development of a country’, International Journal of Multidisciplinary Research and Development 5(8), 100–107.
Balloch, A., Nicolae, A. & Philip, D., 2015, ‘Stock market literacy, trust, and participation’, Review of Finance 19(5), 1925–1963. https://doi.org/10.1093/rof/rfu040
Bank of Tanzania, 2018, Annual Report, Director of Economic Research and Policy, Bank of Tanzania, Dar es Salaam.
Bayer, P.J., Bernheim, B.D. & Scholz, J.K., 1996, The effects of financial education in the workplace: Evidence from a survey of employers, NBER Working paper no. 5655, National Bureau of Economic Research, Cambridge, MA.
Bernheim, B.D., Garrett, D.M. & Maki, D.M., 2001, ‘Education and saving: The long-term effects of high school financial curriculum mandates’, Journal of Public Economics 80(3), 435–465. https://doi.org/10.1016/S0047-2727(00)00120-1
Campbell, J.Y., 2006, ‘Household Finance’, The Journal of Finance 61(4), 1553–1604. https://doi.org/10.1111/j.1540-6261.2006.00883.x
Cheng, Y-F., Mutuc, E.B., Tsai, F-S., Lu, K-H. & Lin, C-H., 2018, ‘Social capital and stock market participation via technologies: The role of households’ risk attitude and cognitive ability’, Sustainability 10(6), 1904. https://doi.org/10.3390/su10061904
Cole, S. & Shastry, K., 2009, Smart money: The effect of education, cogni-tive ability, and financial literacy on financial market participation, Working paper 09-071, Harvard Business School, Boston, MA.
Dillen, N. & De Lille, O., 2018, ‘Stock market participation in the European Union’, Master of Science in de Handelswetenschappen, Universiteit Gent.
Duflo, E. & Saez, E., 2003, ‘The role of information and social interactions in retirement plan decisions: Evidence from a randomized experiment’, Quarterly Journal of Economics 118(3), 815–842. https://doi.org/10.1162/00335530360698432
Financial Engines, 2018, ‘Financial Engines reports fourth quarter and full year 2017 financial results’, Business Wire, viewed n.d., from https://www.businesswire.com/news/home/20180222006162/en/Financial-Engines-Reports-Fourth-Quarter-and-Full-Year-2017-Financial-Results.
Financial Inclusion Report, 2017, ‘Financial inclusion insights, applied research for digital financial inclusion’, Tanzania Wave 5 Report, Fifth Annual FII Tracker Survey, July – August 2017, Financial Inclusion Insights, Dar es Salaam.
Financial Sector Regulators, 2019, The Kenya financial sector stability report, 2018, Issue no. 10.
Fernandes, D., Lynch, J.G. & Netemeyer, R.G., 2014, ‘Financial literacy, financial education and downstream financial behaviors?’, Management Science 60(8), 1861–1883. https://doi.org/10.1287/mnsc.2013.1849
Fohlin, C., 1999, ‘Capital mobilisation and utilisation in latecomer economies: Germany and Italy compared’, European Review of Economic History 3(2), 139–174. https://doi.org/10.1017/S1361491699000088
García, R. & Tessada, J., 2013, The effect of education on financial market participation: Evidence from Chile, Superintendencia de Valores y Seguros, Chile, Pontificia Universidad Católica de Chile, EH-Clio Lab UC and Finance UC.
Georgarakos, D. & Pasini, G., 2011, ‘Trust, sociability, and stock market participation’, Review of Finance 15(4), 693–725. https://doi.org/10.1093/rof/rfr028
Gerhard, P., Gladstone, J.J. & Hoffmann, A.O.I., 2018, ‘Psychological characteristics and household savings behavior: The importance of accounting for ltent heterogeneity’, Journal of Economic Behavior & Organization 148, 66–82. https://doi.org/10.1016/j.jebo.2018.02.013
Gliem, J.A. & Gliem, R.R., 2003, ‘Calculating, interpreting and reporting Cronbach’s Alpa reliability coefficient for likert-type scales’, Presented at the midwest research-to-practice conference in adult, continuing, and community education, 8–10th October, The Ohio State University, Columbus, OH.
GraphPad, 2009, Will the real logistic please stand up: The three meanings of logistic, Knowledgebase, Article 1465, San Diego, CA.
Guiso, L. & Jappelli, T., 2005, ‘Awareness and stock market participation’, Review of Finance 9(4), 537–567. https://doi.org/10.1007/s10679-005-5000-8
Guiso, L., Haliassos, M. & Jappelli, T., 2003, ‘Household stockholding in Europe: Where do we stand and where do we go?’, Economic Policy 18(36), 123–170. https://doi.org/10.1111/1468-0327.00104
Gumbo, M. & Sandada, M., 2018, ‘The determinants of stock market participation: Evidence from individual investors in Zimbabwe’, Acta Universitatis Danubius 14(4), 642–654.
Hilgert, M.A., Hogarth, J.M. & Beverly, S.G., 2003, ‘Household financial management: The connection between knowledge and behavior’, Federal Reserve Bulletin 89(7), 309–322.
Hong, H., Kubik, J. & Stein, J., 2004, ‘Social interaction and stock-market participation’, Journal of Finance 59(1), 137–163. https://doi.org/10.1111/j.1540-6261.2004.00629.x
Hsieh, F.Y., 1989, ‘Sample size tables for logistic regression’, Statistics in Medicine 8(7), 795–802. https://doi.org/10.1002/sim.4780080704
IMF, 2018, United Republic of Tanzania Financial system stability assessment, IMF Country report no. 18/346, International Monetary Fund, Washington, DC.
Isaac, J., 2014, Expanding women’s access to financial services, World Bank, Washington, DC, viewed n.d., from https://www.worldbank.org/en/results/2013/04/01/banking-on-women-extending-womens-access-to-financial-services.
Kadoya, Y., Khan, M.S.R. & Rabbani, N., 2017, Does financial literacy affect stock market participation?, Social Science Research Network, viewed 20 October 2017, from https://ssrn.com/abstract=3056562.
Kang, H., 2013, ‘Appropriate design of research and statistical analyses: Observational versus experimental studies’, Korean Journal of Anesthesiology 65(2), 105–107. https://doi.org/10.4097/kjae.2013.65.2.105
Kartik, N., Ottaviani, M. & Squintani, F., 2016, ‘Credulity, lies, and costly talk’, Journal of Economic Theory 134(1), 93–116. https://doi.org/10.1016/j.jet.2006.04.003
Klapper, L., Lusardi, A. & Van Oudheusden, P., 2015, Financial literacy around the world: Insights from the standard and poor’s ratings services global financial literacy survey, McGraw Hill Financial, viewed 21 December 2019, from https://responsiblefinanceforum.org/wp-content/uploads/2015/12/2015-Finlit_paper_17_F3_SINGLES.pdf.
Kulkarni, U. & Deepa, R.H., 2019, ‘A study of women and stock market – Awareness and participation’, International Journal of Multidisciplinary 4(2), 366–367.
Lusardi, A. & Mitchell, O.S., 2006, Financial literacy and planning: Implications for retirement wellbeing, Pension Research Council working paper no. 1, Cambridge, MA.
Lusardi, A. & Mitchell, O.S., 2007a, ‘Financial literacy and retirement preparedness: Evidence and implications for financial education’, Business Economics 42(1), 35–44. https://doi.org/10.2145/20070104
Lusardi, A. & Mitchell, O.S., 2007b, ‘Baby Boomer retirement security: The roles of planning, financial literacy, and housing wealth’, Journal of Monetary Economics 54(1), 205–224. https://doi.org/10.1016/j.jmoneco.2006.12.001
Lusardi, A. & Mitchell, O.S., 2011, ‘Financial literacy around the world: An overview’, Journal of Pension Economics and Finance 10(4), 497–508. https://doi.org/10.1017/S1474747211000448
Lusardi, A. & Mitchell, O.S., 2014, ‘The economic importance of financial literacy: Theory and evidence’, Journal of Economic Literature 52(1), 5–44. https://doi.org/10.1257/jel.52.1.5
Macha, D., 2009, ‘Developing a National Financial Literacy Strategy – Tanzania’, Conference on promoting financial capability and consumer protection, Accra, 8–9th September, Real Sector and Microfinance Department, Bank of Tanzania.
Martin, M., 2007, A literature review on the effectiveness of financial education, Working paper 07-03, Federal Reserve Bank of Richmond, Richmond, VA.
Massele, J., Darroux, C., Jonathan, H. & Fengju, X., 2013, ‘Challenges faced by Dar-es-Salaam stock exchange market in Tanzania’, Research Journal of Finance and Accounting 4(15), 36–42.
Omole, D.A. & Falokun, G.O., 1999, ‘The impact of interest rate liberalization on corporate financing strategies of quoted companies in Nigeria’, Proceedings of the Conference on African Economic Research Consortium (AERC 99), Kenya, p. 52.
Saeed, O., 1971, ‘Marketability of securities as an incentive for voluntary savings: A case study of the Sudan’, Sudan Notes and Records 52, 88–100.
Shahabadi, A. & Jafari, M., 2017, ‘The role of mobilization of capital market financial resources in order to restitance of Iran economy from achieving sustained and steady economic growth channel’, International Journal of Resistive Economics 5(1), 48–60.
Sindambiwe, P., 2014, ‘Financial literacy, stock market awareness and capital market participation of an emerging stock market’, International Journal of Multidisciplinary Approach and Studies 1(5), 366–406.
Stango, V. & Zinman, J., 2008, ‘Exponential growth bias and household finance’, The Journal of Finance 64(6), 2807–2849. https://doi.org/10.1111/j.1540-6261.2009.01518.x
Stock, J.H. & Watson, M.W., 2007, Introduction to econometrics, Pearson/Addison Wesley, Boston, MA.
Van Rooij, M., Lusardi, A. & Alessie, R., 2011, ‘Financial literacy and stock market participation’, Journal of Financial Economics 101(2), 449–472. https://doi.org/10.1016/j.jfineco.2011.03.006
Yamane, T., 1967, Statistics: An introductory analysis, 2nd edn., Harper and Row, New York, NY.
Yao, R., Hanna, S.D. & Montalto, P.C., 2003, ‘The capital accumulation ratio as an indicator of retirement adequacy’, Journal of Financial Counseling and Planning 14(2), 1–11. https://ssrn.com/abstract=2260472.
Yoong, J., 2011, ‘Financial illiteracy and stock market participation: Evidence from the RAND American life panel’, in O.S. Mitchell & A. Lusardi (eds.), Financial literacy: Implications for retirement security and the financial marketplace, pp. 76–97, Oxford University Press, Oxford.
Ziorklui, S.Q., 2001, Capital market development and growth in sub-Saharan Africa: The case of Tanzania, Discussion paper no. 79, African Economic Policy.
|