Abstract
Orientation: This article is part of an ongoing research project on various aspects of employee productivity in the South African workplace.
Research purpose: The aim of this article is to determine firm-based employee productivity impacts as a result of employee remuneration inequalities (excess-remuneration and under-remuneration) in the South African workplace.
Motivation for the study: The study focuses on understanding the impact and magnitude of employee remuneration inequalities on employee productivity in a unionised South African workplace.
Research design: The article adopts two distinct estimation models. The aim of the additive multivariate linear estimation model is to determine the sign and the significance of the impact of both under- and excess-remuneration levels on employee productivity when employee characteristics such as levels of training, work experience and managerial involvement are considered. The second model is a fixed-effect panel data estimation where the full sample set of the relevant firm-based data is used. The aim of the panel data estimations is to estimate the robustness of the additive multivariate linear estimates. The manufacturing industry of Gauteng has been chosen as the case study, given the importance of this industry, in the gross geographical product of Gauteng province and the availability of firm-based data.
Main findings: Estimation results indicate a strong and significant negative impact of under-remuneration on employee productivity levels. Excess-remuneration levels have a small positive impact on employee productivity levels.
Practical/managerial implications: The estimations indicate the necessity to eliminate remuneration inequalities and opt for equalised remuneration structures for similar occupations in the market to enhance employee productivity levels.
Contribution/value-added: The study contributes to our understanding of the impact of remuneration inequalities for similar occupations on employee productivity.
Keywords: remuneration gap; fixed-panel data estimations; the Sen index; employee productivity; International Standard Classification of Occupations (ISCO-88); additive multivariate linear model.
Introduction
The aim of this article is to determine the impact of employee remuneration inequalities (under-remuneration and excess-remuneration) on employee productivity when firm-based data are used.
The South African workplace is highly unionised and it is hypothesised that remuneration inequalities (especially under-remuneration), which are derived from comparable average industry remuneration levels for particular job levels, would have a strong impact on employee productivity in the workplace. Information on the employee remuneration levels paid by different firms in an industry is rapidly transmitted in a unionised working environment. A unique firm-based estimation methodology is applied in this article where the impact of positive and negative remuneration inequalities on employee productivity is determined.
The article is a follow-up on a study that was conducted on the relationship between remuneration gaps and employee productivity in which employee remuneration gap–employee productivity coefficients were estimated to determine the existence of diminishing marginal employee productivity after some optimal employee remuneration gap level (Van Zyl 2010). It is part of an on-going research project on various aspects of employee productivity in the South African workplace. Research thus far has covered various aspects of firm-based employee productivity. These are, (1) remuneration dispersion (Van Zyl 2010), (2) different age-skill categories (Van Zyl 2013a), (3) qualifications and training levels (Van Zyl 2013b), (4) employee diversity (Van Zyl 2014), (5) incentive schemes (Van Zyl 2015), (6) non-unionised participation platforms (Van Zyl 2016), (7) in-house training (Van Zyl 2017), (8) employee migration to smaller firms (Van Zyl 2019) and (9) the implementation of new technology (Van Zyl 2020).
The structure of the article is as follows: In the literature review, the three major approaches on the link between remuneration inequalities and employee productivity are discussed. The literature review is followed by the research design where the hypotheses are stated, the research approach and method are listed, the data requirements explained, and the model specification presented. The third section captures the estimation results and a detailed discussion thereof. A conclusion constitutes the final section of the article.
Literature study
The literature review focusses specifically on a brief overview of the concepts and measurement methodologies of the link between remuneration inequalities and employee productivity.
The link between employee remuneration inequalities (under-remuneration or excess-remuneration) and employee productivity is well documented in the literature (Agell & Lommerud 1993; Akerlof & Yellen 1986, 1988; Bandiera, Barankay & Rasul 2007; Bazzi, Egger & Muendler 2015; Bester & Petrakis 2003; Bivens & Mishel 2015; Charness & Kuhn 2007; Cohn, Fehr & Gotte 2010; Faggio, Salvanes & Van Reenen 2007; Furman & Orszag 2018; Helpman, Itskhoki & Redding 2008; Hibbs & Locking 2000; Liu 2002; Mahy, Rycx & Volral 2011; Rogers & Vernon 2002; Van Biesebroeck 2015). In main, these studies employ aggregate conventional econometric models to investigate published data sets on the link between employee remuneration inequalities and employee productivity. Studies that apply firm-based data, especially in the South African context, could not be found. The research findings in general indicate (1) a negative relationship between under-remuneration and employee productivity and (2) a positive relationship between excess-remuneration and employee productivity. Research findings also indicate a bigger negative magnitude of the impact of under-remuneration on employee productivity when compared to the positive magnitude of the impact of excess-remuneration on employee productivity.
There are basically three main approaches in the literature on the measurement methodologies of the link between remuneration inequalities and employee productivity. The first approach (Akerlof & Yellen 1986, 1988) posits that employee remuneration levels are determined by employee productivity levels (and not the other way around) in the workplace. The cluster of the various employee remuneration levels should reflect the marginal efficiency of employee productivity. Employee remuneration inequalities are not captured as independent variables in econometric estimation models. The argument is that to achieve greater collective employee productivity levels (as a result of improved skill levels, higher levels of training, and the implementation of new technologies), large degrees of intra-firm and inter-industry remuneration dispersions should be avoided. Smaller levels of remuneration inequalities reflect more realistic marginal efficiencies of employee productivity.
The second approach (Bester & Petrakis 2003; Capelli & Chauvin 1991; Georgiev & Hendriksen 2020; Helpman et al. 2008) argues that it is difficult to match employee remuneration levels to employee marginal efficiencies given the complexities of the employer–employee relationship. These complexities include aspects such as skill levels, job experience, the implementation and introduction of new technologies in the workplace, and matching employee productivity to employee remuneration. This approach argues for the control of employee diversity variables in the econometric modelling of the net employee productivity effects of remuneration inequalities in the workplace. Owing to an imperfect flow of information in the market on aspects such as employee productivity and employee remuneration levels (for different job descriptions), this approach argues for a non-linear distribution of employee remuneration in the same job categories between firms and industries. Weiss (1990) and Groshen (1991) also indicate that the imperfect flow of information in the job market may create a situation where firms introduce employee remuneration inequalities (especially excess-remuneration levels) to increase employee productivity levels. This description is linked to the concept known as ‘shirking’. The basic argument is that employees who are paid a remuneration level higher than the same comparable average remuneration in the industry, will be incentivised to improve their productivity levels to avoid dismissal. The consensus of research findings in this approach is that higher employee productivity levels require positive remuneration inequality (excess-remuneration) in the workplace. Firms with high levels of remuneration inequalities (especially under-remuneration) have lower employee productivity levels because of the perception that employee effort is not matched by a comparable ‘fair’ remuneration level. This is true for intra-firm and inter-industry comparisons. Bandiera et al. (2007) are of the opinion that employee characteristics are important when the effect of negative remuneration inequalities is considered. The existence of negative remuneration inequalities will have a stronger negative impact on the productivity levels of employees with higher skill and training levels compared to less skilled and less trained employees.
The third approach (Bazzi et al. 2015; Ellis 2003; Liu 2002) argues that market dynamics will limit employee remuneration inequality levels in the workplace. The argument is that as a result of the effective flow of information in job markets and the strength of labour union participation, firms that prefer to pay non-market-related remuneration levels (especially under-remuneration levels) will not be able to attract qualified employees. Furman and Orszag (2018) indicate that more intense levels of union activity in the industry do create greater levels of job and remuneration security for their members and will have a stronger negative impact on employee productivity in the case of perceived negative remuneration inequalities in the industry. The argument is that there is less negative remuneration inequality in unionised firms or industries than in non-unionised firms or industries. It is also argued that lower levels of negative remuneration inequality in the workplace, because of greater levels of union activity, have a reduced negative impact on employee productivity. The findings of Liu (2002) indicate that employee diversity variables such as job experience, training levels and participation in managerial positions, and the strength of remuneration inequalities need to be included when the impact of remuneration inequalities on employee productivity is estimated. Some researchers (Bivens & Mishel 2015, for example) are of the opinion that economic conditions would determine the magnitude of the impact of remuneration inequality on employee productivity. In the case of difficult economic conditions, the impact of remuneration inequality (especially under-remuneration) on employee productivity will be limited, as employees are reluctant to lose their jobs. The reverse argument is also true. Given the more effective flow of information in the workplace, the perception of what a ‘fair’ remuneration level for a comparable job description in the industry is, will have an impact on employee productivity. Norsworthy and Zabala (1985) noted that employees can easily determine a ‘fair’ level of remuneration for comparable job levels in the industry. Negative remuneration inequality (under-remuneration) will have a negative impact on employee productivity. The increase in intra-firm and inter-industry managerial ratios could create higher levels of remuneration inequality (Rogers & Vernon 2002; Van Biesebroeck 2015).
The article applies the methodology of the third approach, more specifically the measurement methodology of Liu (2002).
Research design
The research design comprises the hypotheses to be tested, a detailed outlay of the research approach, followed by a step by step explanation of the model specification. This section is concluded with an outline of the data requirements for the econometric modelling.
Hypotheses
The three hypotheses tested in the article are as follows:
H1: For a firm-based data set, the magnitude of the negative impact of under-remuneration on employee productivity is greater than the magnitude of the positive impact of excess-remuneration on employee productivity.
H10: For a firm-based data set, the magnitude of the negative impact of under-remuneration on employee productivity is not greater than the magnitude of the positive impact of excess-remuneration on employee productivity.
H2: For a firm-based data set, greater levels of investment in new technology and equipment will reduce the negative impact on employee productivity because of under-remuneration.
H20: For a firm-based data set, greater levels of investment in new technology and equipment will not reduce the negative impact on employee productivity because of under-remuneration.
H3: For a firm-based data set, the negative employee productivity effects in the case of under-remuneration are greater in cases of higher levels of work experience and better-trained employees.
H30: For a firm-based data set, the negative employee productivity effects in the case of under-remuneration are not greater in cases of higher levels of work experience and better-trained employees.
Research approach
An adaptive version of the estimation models of Liu (2002) has been applied in this article to cater for the South African workplace. The following estimations methodologies are applied in the article:
- A Sen index to measure the degree of under-remuneration for the sample period per major code of occupations 71 and 72. An index value between 0.5 and 1 is an indication of a strong degree of under-remuneration whilst an index value between 0 and 0.5 is indicative of a weak degree of under-remuneration.
- A modified Sen index for levels of excess-remuneration for the sample period. This index is constructed to measure the degree of excess-remuneration per major code of occupations 71 and 72. An index value between 0.5 and 1 is an indication of a strong degree of excess-remuneration whilst an index value between 0 and 0.5 is indicative of a weak degree of excess-remuneration.
- A Pearson correlation matrix. The aim of the Pearson matrix is to determine the strength and the sign of the bivariate relationship between the remuneration inequality variables (more specifically the degree of under-remuneration, the degree of excess-remuneration and the coefficient of variation from the average remuneration levels) and the expected impact on employee productivity. A high bivariate relationship between the remuneration inequality variables and the expected impact on employee productivity serve as a strong motivation to apply an additive multivariate linear estimation model.
- An additive multivariate linear estimation model. The aim is to include quarterly data series of average values for capital expenditure, the average number of employees in major codes 71 and 72, the average remuneration per job category, the degree of under-remuneration and excess-remuneration and employee characteristics such as the average years of training, average job experience and average management participation rates when the impact of remuneration inequalities on employee productivity are measured.
- Fixed-effect panel data estimations. The full sets of firm data are applied to test for the robustness of the results of the additive multivariate linear model. A greater level of similarity between the estimation results of the additive multivariate linear estimation model and the estimation results of the fixed-effect panel data estimation will confirm the robustness of the additive multivariate linear model estimates.
- A regression analysis to determine the contribution of aggregate levels of under-remuneration and excess-remuneration to the variation in employee remuneration and also at the same time the strength of other factors that would contribute to the variation in employee remuneration.
The application of both the additive multivariate linear model and the fixed-effect panel data estimation is based on the mathematical and econometric construct of relative under-remuneration, the measurement of relative excess-remuneration, the inclusion of a Sen index for under-remuneration, modified Sen index for excess-remuneration and various employee diversity aggregates.
The estimation results are interpreted and the implications thereof on employee productivity are explained.
Model specification
The estimation steps underpinning the model construct applied in the article are explained in the following paragraphs.
The first step of the estimation process is the estimation of the Pearson correlation coefficients that will indicate the strength and sign of the bivariate relationships between the different remuneration inequality variables (specifically the degree of under-remuneration, the degree of excess-remuneration and the degree of variation for employee remuneration) as an indication of the need for a multivariate analysis of the impact on employee productivity. As mentioned in the research approach, strong bivariate relationships between the different variables are indicative of the need for multivariate estimations to determine the impact of the different variables of remuneration inequalities on employee productivity. For the Pearson correlation coefficient estimation, the real average employee output vector (LnRPH) is treated as the dependant variable, and the Sen index vector for the degree of aggregate under-remuneration (LnSIUR), the modified Sen index vector for excess-remuneration (LnSIER) and the coefficient of variation vector for employee remuneration (LnVER) as the dependant variables. This is done for both major codes of occupation 71 and 72 of International Standard Classification of Occupations (ISCO-88).
The second step is to perform an additive multivariate regression analysis (Equation 1) to estimate the net effects of under-remuneration and excess-remuneration on employee productivity. The data series for the variables are constructed as quarterly average values for the sample group of firms over the sample period. The following equation is expressed in log-linear format for both sides of the equation:
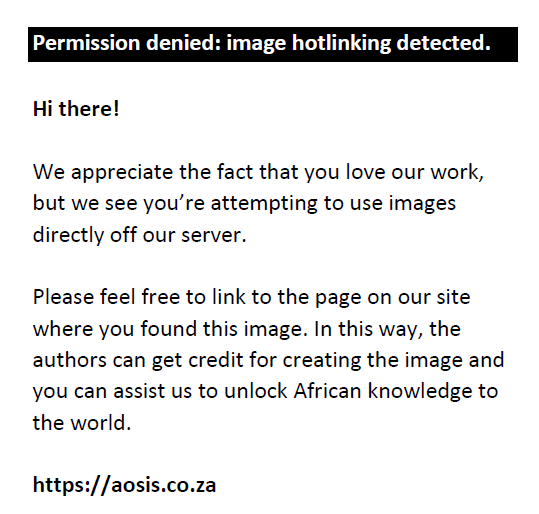
The dependant variable LnQ represents the real average output per employee and is the proxy for employee productivity changes. Amongst independent variables, αLnK represents the vector of associated capital expenditure, βLnL represents the vector of the average level of employees per ISCO major job categories 71 and 72, δ represents the employee productivity impact of excess-remuneration (LnER) and under-remuneration (LnUR), λ represents the employee diversity impact – namely years of training (LnATE), the management participation rate (LnMP) and job experience (LnAWE) – and ε is the error term.
The interpretation of especially δ1, δ2, λ1, λ2 and λ3 is important. If δ1 < 0, the aggregate level of relative under-remuneration has a negative effect on employee productivity. If δ2 > 0, the aggregate level of relative excess-remuneration has a positive impact on employee productivity. A negative sign for λ is indicative of a negative employee productivity impact and a positive sign is indicative of a positive employee productivity impact. The greater the magnitude of the negative or positive sign the greater the impact on employee productivity.
The inclusion of the capital vector (αLnK) caters not only for real capital expenditure but also for the different levels of new technology that might impact on employee productivity.
The third step is a fixed-effect panel data estimation. The aim is to determine the robustness of the additive multivariate regression estimates by controlling for the level of technology and to eliminate the problem of auto-correlated error terms across the sample period for each of the major codes of occupation 71 and 72 of ISCO-88. The full firm-based data sets are applied in the fixed-effect panel data estimation. The impact of (1) real investment spending in new technology (LnACW), (2) the levels of training (LnATE), (3) the levels of work experience (LnAWE), (4) the degree of under-remuneration (LnSIUR), (5) the degree of excess-remuneration (LnSIER), (6) the coefficient of variation from average remuneration levels (LnVER) and (7) the percentage participation of employees in managerial positions (LnMP) on employee productivity are estimated for the full data set. Positive estimates for LnACW are an indication that greater capital investment in new technologies will reduce the negative employee productivity levels because of under-remuneration. Negative estimates for both the level of training (LnATE) and the level of work experience (LnAWE) are indicative of a negative impact on employee productivity (because of under-remuneration) for employees that have higher levels of training and greater levels of work experience. Negative estimates for LnSIUR indicate that a higher degree of under-remuneration will have a negative impact on employee productivity whilst positive estimates for LnSIER indicate a positive impact on employee productivity for a higher degree of under-remuneration levels. Positive estimates for LnMP are an indication that higher levels of managerial participation limit the impact of both under-remuneration and excess-remuneration on employee productivity. Negative estimates for LnVER indicate that larger deviations from the average remuneration levels, especially for under-remuneration levels, will generate additional negative impacts on employee productivity.
The fourth step is to run a regression in which the coefficient of variation (LnVER) from average remuneration levels is the dependant variable and the degree of under-remuneration and excess-remuneration (LnSIUR and LnSIER) are the independent variables. As mentioned in the research design, the aim of this estimation is to determine the strength of aggregate levels of under-remuneration and excess-remuneration to the variation in employee remuneration. The R2 will explain the impact strength of aggregate levels of under-remuneration and excess-remuneration on the variation in employee remuneration. The higher the R2, the greater the impact of aggregate levels of under-remuneration and excess-remuneration on the variation in employee remuneration. The lower the R2, the higher the impact of other factors on the variation in employee remuneration.
Data requirements
The manufacturing industry of Gauteng province (given the availability of firm-based data and the importance of the manufacturing industry in the gross geographic product of the province) is used as a case study to capture the employee productivity effects because of remuneration inequalities (under-remuneration and excess-remuneration). The sample set of 115 firms covers a variety of sub-sectors of the manufacturing industry and is deemed to be statistically significant. The data series of the firms are indexed to protect the confidentiality of the data. All the data collected from the firms are secondary data. Ethical clearance was obtained to use the firm-based data (code 20SECO17). This article uses the ISCO-88 major groups 71 (craft and building trades employees) and 72 (metal, machinery and related trades employees). More specifically, it uses digits 712 (building frame and related trades employees), 713 (building finishers and related trades employees), 714 (painters, building structure cleaners and related trades employees), 721 (metal moulders, welders, sheet metal employees, structural-metal prepares and related trades employees) and 722 (black smiths, tool-makers and related trades employees). The average remuneration level per specified ISCO-88 major job digits for the sample group of firms is calculated. Employee remuneration levels below this average are deemed to be under-remuneration whilst remuneration levels above the average are deemed to be excess-remuneration.
The sample period is 2011–2016 (the required data sets are converted to a quarterly basis). Only real values (catering for inflation for the sample period data) are applied. For each firm in the sample group, the following required data sets apply for the sample period:
- average real annual production converted into the Rand-value of quarterly output per employee
- real quarterly remuneration for each major code of occupation 71 and 72
- real quarterly spending on capital per employee
- mean years of training per major code of occupations 71 and 72 (in quarterly series)
- mean years of working experience per major code of occupations 71 and 72 (in quarterly series)
- percentage number of employees in management positions per major codes of occupations 71 and 72 (in quarterly series).
Construct, (1) quarterly data series for levels of under-remuneration and excess-remuneration for the sample group over the sample period per major code of occupations 71 and 72, (2) a Sen index for aggregate levels of under-remuneration for the sample period (in quarterly series), (3) a modified Sen index for levels of excess-remuneration (in quarterly series), (4) data series for the coefficient of variation from the average employee remuneration levels for both major codes of occupation (71 and 72), (5) a data series for the mean percentage of employees in managerial positions over the sample period per major codes of occupation 71 and 72 (in quarterly series).
Estimation results
The estimation results (and the explanation thereof) of the Pearson correlation coefficient matrix, the additive multivariate linear modelling, the fixed-effect panel data modelling, and the coefficient of variation modelling are presented in this section.
The Pearson correlation estimates for the digit codes of occupation 71 and 72 are presented in Tables 1 and 2.
TABLE 1: Pearson correlation coefficients (N = 115); major code 71 (digits 712, 713 and 714). |
TABLE 2: Pearson correlation coefficients (N = 115); major code 72 (digits 721, 722 and 723). |
The dependant variable (LnRPH) has a correlation of −0.64 with the Sen index (LnSIUR) in the case of major code 71 and a correlation of −0.57 for major code 72. These are relatively high negative coefficients and are an indication of a strong bivariate relationship between employee productivity and the degree of under-remuneration for both major codes of occupation. A high level of under-remuneration inequality has a significant negative impact on employee productivity (as measured by real employee production values). This result is expected given the high levels of unionisation in the workplace.
The correlation between the dependent variable LnRPH and the lagged modified Sen index (LnSIER) is relatively weak positive for both major codes of occupation 71 and 72. It indicates a weak positive bivariate relationship between employee productivity and the degree of excess-remuneration. This result confirms similar international results that indicate the limited positive impact that excess-remuneration will have on employee productivity.
Under-remuneration has a relatively high negative correlation with excess-remuneration (−0.59 for major code 71 and −0.52 for major code 72). This result implies that firms that remunerate a section of their employees at rates lower than the comparable average remuneration level, given certain employee characteristics (training, age and experience), are also less likely to over-remunerate a section of their employees because of certain employee characteristics (training, age and experience).
There are low positive correlations between the Sen index for under-remuneration and the coefficient of variation for employee remuneration (0.28 for digit 71 and 0.38 for digit 72) and the Sen index for excess-remuneration and the coefficient of variation for employee remuneration (0.13 for digit 71 and 0.38 for digit 72). These results indicate that the levels of remuneration inequality will react slowly to changes in the variation of employee remuneration.
The results of the Pearson correlation matrix are a clear indication that a multivariate estimation is needed to establish a clearer link between remuneration inequalities and employee productivity.
The estimation results of the additive multivariate regression analysis (Equation 1) are listed in Table 3.
TABLE 3: Estimation results of the additive multivariate regression analysis. |
The magnitudes and signs of the estimated coefficients for the sample group of firms are in general similar for both digits 71 and 72 occupations.
Irrespective of the possibilities of under- and excess-remuneration levels, real capital expenditure has a positive impact on employee productivity (α). For the average firm-based sample data set, it implies that the net positive employee productivity levels generated by greater levels of investment in new technologies and equipment would counter negative employee productivity levels because of under-remuneration.
The positive sign of parameter β is an indication of the net positive employee productivity effects generated by a more efficient employee component even if under-remuneration levels are experienced. It is important to note that in relative terms the positive magnitudes for both major job categories 71 and 72 are small.
The estimation results for parameter (δ1) indicate a net relatively strong negative impact on employee productivity for levels of under-remuneration. Given the high level of unionisation in the labour market, this estimation result was expected.
The estimation results for parameter (δ2) indicate a net positive impact on employee productivity for levels of excess-remuneration. It is important to note that the magnitude of this positive impact on employee productivity is much lower than the magnitude of the negative impact on employee productivity in the case of under-remuneration.
Both the estimates for parameters λ1 and λ3 are negative. These results indicate that the net negative employee productivity effects of under-remuneration are especially strong for the category of employees that have high levels of work experience and training. This is true for both major job categories 71 and 72.
The positive estimates for parameter λ2 indicate that higher percentage levels of managerial positions within the two major codes of occupations would create a net positive effect on employee productivity (the percentage positive impact of excess-remuneration on employee productivity would exceed the percentage negative impact of under-remuneration).
The estimation results of the fixed-effect panel data modelling are listed in Table 4. As previously stated, the fixed-effect panel data specification is applied to determine the robustness of the additive multivariate linear model when the full sample data set is incorporated.
TABLE 4: Estimation results of the fixed-effect panel data estimation. |
The magnitude and signs of the fixed-effect panel data estimation results are relatively similar for both major codes of occupation.
For the full data set the estimation results for parameter LnACW indicate net positive impacts on employee productivity because of real investment expenditure in new technology and equipment (irrespective of under-remuneration or excess-remuneration levels). This result is in line with the estimation results of the additive linear model estimation results.
For the full data set, the estimates for higher levels of training (LnATE) and work experience (LnAWE) are both negative. These results indicate net negative impacts on employee productivity when under-remuneration levels, excess-remuneration levels and the two Sen indexes are included in the estimations. These results are in line with the estimation results in the additive multivariate linear model. The results confirm that the negative impact on employee productivity created by under-remuneration is pertinent for the more highly trained and experienced group of employees.
For the full sample set, the estimations for the Sen index (LnSIUR) indicate that under-remuneration levels have a strong negative impact on employee productivity levels (as measured by the real change in the value of production levels) for both major codes 71 and 72. The estimates indicate that a 1% increase in the Sen index reduces the real value of output by 0.27% for digit 71 occupations and 0.15% for digit 72 occupations. The estimations for the modified Sen index (LnSIER) indicate that excess-remuneration levels have a weaker positive impact on employee productivity levels. For excess-remuneration, a 1% increase in the Sen index increases real output per employee by 0.11% for digit 71 occupations and 0.10% for digit 72 occupations. In general, these estimation results confirm the stronger magnitude of the negative employee productivity effects (because of under-remuneration) when compared to the positive impacts on employee productivity (because of excess-remuneration).
For the full sample set, the estimates for the coefficient of variation (LnVER) for employee remuneration are negative (for both major codes of occupation). The results confirm that larger deviations from the average remuneration level for the full sample of firms, especially in the case of under-remuneration, have additional negative impacts on employee productivity. The magnitude of any positive employee productivity impacts because of greater variation in excess-remuneration is smaller than the magnitude of the negative impacts on employee productivity because of the larger variations in under-remuneration levels.
The estimates for the percentage participation of employees in managerial positions (LnMP) are positive but the magnitude of the estimates is weak. The implication of the estimates is that a greater percentage of employee participation in managerial positions will limit the employee productivity effects generated by excess- and under-remuneration levels.
The estimates of the simple regression to determine the magnitude of variation in employee remuneration, because of aggregate levels of under-remuneration and excess-remuneration, are presented in Table 5. In this simple linear regression, LnVER is the dependent variable and LnSIUR and LnSIER are the independent variables.
TABLE 5: Regression results of the coefficient of variation vector for employee remuneration levels. |
The estimates indicate that for both major codes 71 and 72, under-remuneration (LnSIUR) has a sizable and significant impact on the variation in employee remuneration (LnVER) but excess-remuneration (LnSIER) has a lesser impact on the variation in employee remuneration. The R2 is significant and indicates that that 54.1% (major code 71) and 57.2% (major code 72) of the variation in employee remuneration, respectively, are explained by the aggregate levels of under-remuneration and excess-remuneration. Only 46.9% (major code 71) and 42.8% (major code 72) of the variation in employee remuneration, respectively, reflects other factors that would contribute to wage inequalities. In conclusion, under-remuneration is a major contributor to remuneration inequalities.
The results of both the additive multivariate regression and the fixed-effect panel data estimations confirm the acceptance of hypotheses H1, H2 and H3 and the rejection of the null-hypotheses H10, H20 and H30.
Conclusion
The aim of this article is to determine the impact of negative (under-remuneration) and positive (excess-remuneration) remuneration gaps on employee productivity.
To understand the estimation results, the strongly unionised nature of the South African workplace and the efficiency of the job market to gather data, transmit and disseminate information, must be recognised. This is especially true for the existence of employee remuneration inequalities.
For all the estimations (for both models) that were done, there is a clear indication that the existence of under-remuneration in the workplace has a relatively significant negative impact on employee productivity. The Pearson correlation coefficients for both the major codes of occupation (71 and 72) indicated a strong bivariate relationship between employee productivity and the degree of under-remuneration. The additive multivariate regression estimates, and the fixed-panel data estimates also indicate the relatively significant negative relationship between employee productivity and levels of under-remuneration. The negative relationship is especially significant for employee groupings in the categories of higher levels of work experience and training. The expectation that excess-remuneration would have a positive impact on employee productivity did materialise. The findings of both estimation models are in line with some international studies that clearly indicates a strong negative impact of levels of under-remuneration on employee productivity and a weak positive impact of excess-remuneration on employee productivity.
It is important to note that the study concludes that greater levels of investment in new technologies and equipment do negate the inequality in employee remuneration, especially in the case of under-remuneration. Reduced levels of significant investment in new technology and equipment could have increasingly negative impacts on employee productivity if negative remuneration inequalities are prevalent.
Further studies in this regard could include industry and geographical differences on the impact of remuneration inequalities on employee productivity and the impact of the introduction of other employee diversity aggregates such as gender and race.
Acknowledgements
Competing interests
The author has declared that no competing interest exists.
Author’s contributions
This is a single-authored article and it meets the criteria for authorship as outlined by the policy.
Ethical considerations
School of Economics Research Ethics Committee at the University of Johannesburg, 20SECO17.
Funding Information
This study received no specific grants from any institution or individual.
Data availability
The study worked with secondary firm-based data sources which are indexed. See the ethical clearance document.
Disclaimer
The views and opinions expressed in this article are those of the author and do not reflect the official policy or position of any affiliated agency of the author.
References
Agell, J. & Lommerud, K.E., 1993, ‘Egalitarianism and growth’, Journal of Economics 95(4), 559–579. https://doi.org/10.2307/3440911
Akerlof, G.A. & Yellen., 1986, Efficiency wage model of the labor market, Cambridge University Press, New York, NY.
Akerlof, G.A. & Yellen, J.L., 1988, ‘Fairness and unemployment’, American Economic Review 78(2), 44–49.
Bandiera, O., Barankay, I. & Rasul, I., 2007, ‘Incentives for managers and inequality among workers: Evidence from a firm-level experiment’, Quarterly Journal of Economics 122(2), 729–773. https://doi.org/10.1162/qjec.122.2.729
Bazzi, S., Eggar, H. & Muendler, M.A, 2015, Labor productivity and wage inequality, Working Paper, Boston University, Boston, MA.
Bester, H. & Petrakis, E., 2003, ‘Wages and productivity growth in a competitive industry’, Journal of Economic Theory 109(1), 52–69. https://doi.org/10.1016/S0022-0531(02)00037-6
Bivens, J. & Mishel, L., 2015, ‘Understanding the historic divergence between productivity and a typical worker’s pay: Why it matters and why it is real’, EPI Briefing Papers 406, 1–5, Economic Policy Institute (EPI), Washington.
Capelli, P. & Chauvin, K., 1991, ‘An interplant test of the efficiency wage hypothesis’, Quarterly Journal of Economics 106(3), 769–787. https://doi.org/10.2307/2937926
Charness, G. & Kuhn, P., 2007, ‘Does pay inequality affect worker effort? Experimental evidence’, Journal of Labor Economics 25(4), 693–723. https://doi.org/10.1086/519540
Cohn, A., Fehr, E. & Gotte, L., 2010, ‘Fair wages and effort: Evidence from a field experiment’, Management Science 61(8), 1777–1794. https://doi.org/10.1287/mnsc.2014.1970
Ellis, H., 2003, ‘On the impact of inequality on productivity growth in the short and long term: A synthesis’, Canadian Public Policy 29, 65–86. https://doi.org/10.2307/3552277
Faggio, G., Salvanes, K. & Van Reenen, J., 2007, The evolution of inequality in productivity and wages: Panel data evidence, NEBR Working Paper 13351, National Bureau of Economic Research (NBER), Cambridge.
Furman, J. & Orszag, P., 2018, Slower productivity and higher inequality: Are they related?, PIIE Working Paper WP18-4, Peterson Institute for International Economics (PIIE), Washington.
Georgiev, B. & Henriksen, J.J., 2020, ‘Within-firm wage inequality and firm-level exports’, Review of International Economics 28(1), 1–34. https://doi.org/10.1111/roie.12439
Groshen, E.L., 1991, ‘Sources of intra-industry wage dispersion: How much do employers’ matter’, Quarterly Journal of Economics 106(3), 869–884. https://doi.org/10.2307/2937931
Helpman, E., Itskhoki, O. & Redding, S., 2008, Wages, unemployment and inequality with heterogeneous firms and workers, NBER Working Paper 14122.
Hibbs, D.A. & Locking, H., 2000, ‘Wage dispersion and productive efficiency: Evidence for Sweden’, Journal of Labor Economics 18(4), 755–782. https://doi.org/10.1086/209976
Liu, J., 2002, ‘Does wage inequality affect labor productivity? Some evidence from manufacturing industries of Taiwan and South Korea’, Asia Pacific Management Review 7(4), 449–476.
Mahy, B., Rycx, F. & Volral, M., 2011, ‘Wage dispersion and firm productivity in different working environments’, British Journal of Industrial Relations 49(3), 460–485. https://doi.org/10.1111/j.1467-8543.2009.00775.x
Norsworthy, J.R. & Zabala, C.A., 1985, ‘Effects of worker attitudes on production costs and the value of capital input’, Economic Journal 95(380), 992–1002. https://doi.org/10.2307/2233260
Rogers, M. & Vernon, G., 2002, Wage inequality and productivity growth: Motivating carrots and crippling sticks, SKOPE Research paper 40, Centre on Skills, Knowledge and Organisational Performance (SKOPE), Oxford.
Van Biesebroeck, J., 2015, How tight is the link between wages and productivity? A survey of the literature, ILO Conditions of Work and Employment Series 54, International Labor Organization (ILO), Geneva.
Van Zyl, G., 2010, ‘Does employee remuneration dispersion in the South African economy enhance labour productivity? The Gauteng manufacturing industry as a case study’, Journal of Economic & Financial Sciences 8(1), 1–5. https://doi.org/10.4102/sajhrm.v8i1.286
Van Zyl, G., 2013a, ‘Relative labour productivity contribution of different age-skill categories for a developing economy: The Gauteng province of South Africa as a case study’, South African Journal of Human Resource Management 11(1), 1–8. https://doi.org/10.4102/sajhrm.v11i1.472
Van Zyl, G., 2013b, ‘Positive labour productivity externalities that arises from a post-secondary qualification or training’, Journal of Economic & Financial Sciences 6(3), 761–777. https://doi.org/10.4102/jef.v6i3.257
Van Zyl, G., 2014, ‘Labour productivity and employee diversity in the South African workplace’, Journal of Economic & Financial Sciences 7(2), 451–466. https://doi.org/10.4102/jef.v7i2.150
Van Zyl, G., 2015, ‘Impact of incentive schemes on employee productivity in the South African workplace’, Journal of Economic & Financial Sciences 8(2), 633–647. https://doi.org/10.4102/jef.v8i2.113
Van Zyl, G., 2016, ‘Impact of non-unionised participation platforms on employee productivity in the South African workplace’, Journal of Economic & Financial Sciences 9(1), 93–105. https://doi.org/10.4102/jef.v9i1.31
Van Zyl, G., 2017, ‘Impact of in-house training on employee productivity in the South African workplace’, Journal of Economic & Financial Sciences 10(1), 160–175. https://doi.org/10.4102/jef.v10i1.11
Van Zyl, G., 2019, ‘Employee diversity attributes of productivity and real remuneration spillover impacts of employee migration to smaller firms in the South African workplace’, Journal of Economic and Financial Sciences 12(1), 1–8. https://doi.org/10.4102/jef.v12i1.203
Van Zyl, G., 2020, ‘The impact of new production technology on employee productivity in the South African workplace’, Journal of Economic and Financial Sciences 13(1), 1–8. https://doi.org/10.4102/jef.v13i1.507
Weiss, A., 1990, Efficiency wages, Princeton University Press, Princeton, NJ.
Appendix 1: Descriptive statistics
|